ABSTRACT
Objective: To test a prediction model for sleep apnea based on clinical and sociodemographic variables in a population suspected of having sleep disorders and submitted to polysomnography. Methods: We included 323 consecutive patients submitted to polysomnography because of the clinical suspicion of having sleep disorders. We used a questionnaire with sociodemographic questions and the Epworth sleepiness scale. Blood pressure, weight, height, and SpO2 were measured. Multiple linear regression was used in order to create a prediction model for sleep apnea, the apnea-hypopnea index (AHI) being the dependent variable. Multinomial logistic regression was used in order to identify factors independently associated with the severity of apnea (mild, moderate, or severe) in comparison with the absence of apnea. Results: The prevalence of sleep apnea in the study population was 71.2%. Sleep apnea was more prevalent in men than in women (81.2% vs. 56.8%; p < 0.001). The multiple linear regression model, using log AHI as the dependent variable, was composed of the following independent variables: neck circumference, witnessed apnea, age, BMI, and allergic rhinitis. The best-fit linear regression model explained 39% of the AHI variation. In the multinomial logistic regression, mild apnea was associated with BMI and neck circumference, whereas severe apnea was associated with age, BMI, neck circumference, and witnessed apnea. Conclusions: Although the use of clinical prediction models for sleep apnea does not replace polysomnography as a tool for its diagnosis, they can optimize the process of deciding when polysomnography is indicated and increase the chance of obtaining positive polysomnography findings.
Keywords:
Sleep apnea syndromes; Polysomnography; Sleep apnea, obstructive; Body mass index.
RESUMO
Objetivo: Testar um modelo de predição para apneia do sono a partir de variáveis sociodemográficas e clínicas em uma população com suspeita de distúrbio do sono e submetida à polissonografia. Métodos: Foram incluídos no estudo 323 pacientes consecutivos submetidos à polissonografia por suspeita clínica de distúrbio do sono. Utilizou-se um questionário com questões sociodemográficas e a escala de sonolência de Epworth. Foram medidos pressão arterial, peso, altura e SpO2. A regressão linear múltipla, tendo o índice de apneia-hipopneia (IAH) como variável dependente, foi utilizada para construir um modelo de predição de apneia do sono. A regressão logística multinomial foi realizada para verificar fatores associados de forma independente à gravidade da apneia (leve, moderada ou grave) em comparação à ausência de apneia. Resultados: A prevalência de apneia do sono na população de estudo foi de 71,2%, e foi mais prevalente nos homens que nas mulheres (81,2% vs. 56,8%; p < 0,001). O modelo de regressão linear múltipla, com o log IAH como variável dependente, foi composto pelas seguintes variáveis independentes: circunferência do pescoço, apneia testemunhada, idade, IMC e presença de rinite alérgica. O melhor modelo de regressão linear encontrado conseguiu explicar 39% da variabilidade do IAH. Na regressão logística multinomial, a apneia leve esteve associada com IMC e circunferência do pescoço, e a apneia grave associou-se com idade, IMC, circunferência do pescoço e apneia testemunhada. Conclusões: Modelos de predição clínica para apneia do sono não substituem a polissonografia como ferramenta para o seu diagnóstico, mas podem otimizar sua indicação e aumentar a chance de positividade do exame.
Palavras-chave:
Síndromes da apneia do sono; Polissonografia; Apneia do sono tipo obstrutiva; Índice de massa corporal.
IntroduçãoOs distúrbios respiratórios do sono são definidos como síndromes nas quais a frequência e a intensidade dos eventos encontram-se fisiopatologicamente associadas a sintomas ou desfechos adversos à saúde.
A frequência de apneias e hipopneias é expressa pelo índice de apneia-hipopneia (IAH), que corresponde à soma do número de apneias e hipopneias dividido pelo total de horas de sono. A gravidade é classificada de acordo com o IAH: IAH de 5-15 eventos/h (leve); IAH de 15-30 eventos/h (moderada); e IAH > 30 eventos/h (grave). (1)
Um estudo populacional realizado na cidade de São Paulo (SP), utilizando os critérios diagnósticos da International Classification of Sleep Disorders, em sua segunda versão de 2005, da American Academy of Sleep Medicine, mostrou uma prevalência geral de apneia obstrutiva do sono em 32,8% da população. (2)
Utilizando-se como critério diagnóstico um IAH ≥ 5 eventos/h, a prevalência de apneia do sono em homens atendidos em laboratórios do sono varia de 17-26%. (3)
Um estudo prospectivo sobre sono no estado de Wisconsin, EUA, mostrou que uma elevação de 10% no peso basal do indivíduo prediz um aumento de 32% no IAH (IC95%: 20-45%). (4) Por outro lado, uma redução de 10% no peso inicial diminui em 26% o IAH (IC95%: 18-34%). A apneia do sono também é comum em pacientes com distúrbios ou alterações na estrutura craniofacial. (5)
A fragmentação do sono com despertares no final de um episódio de apneia causa sonolência excessiva em alguns pacientes. Oscilações da descarga simpática relacionadas aos episódios de apneia produzem um padrão de dessaturação da oxi-hemoglobina seguido pela normalização da saturação, podendo provocar lesões em neurônios que promovem o estado de vigília. (6,7) Essas lesões contribuem para distúrbios de aprendizagem e podem ser a base para a sonolência residual que é encontrada em pacientes com apneia do sono mesmo sob tratamento adequado. (5)
A frequência cardíaca e a pressão arterial aumentam em torno de cinco a sete segundos após o final da apneia, coincidindo com o microdespertar e a dessaturação da oxi-hemoglobina. (8,9)
Parece haver uma relação entre a gravidade da apneia e o desenvolvimento de hipertensão arterial, associação essa que persiste mesmo após o controle para variáveis de confusão. (4) Pacientes com apneia do sono grave, não tratados, têm um maior risco de apresentar eventos cardiovasculares em um período de 10 anos de seguimento quando comparados a controles com graus semelhantes de obesidade. (10)
A polissonografia de noite inteira é indicada para o diagnóstico dos diferentes distúrbios do sono, principalmente aqueles respiratórios.(1)
Sistemas portáteis para a monitoração domiciliar do sono estão se difundindo rapidamente no mercado e sendo utilizados principalmente para o diagnóstico de apneia-hipopneia obstrutiva do sono. A Associação Americana de Distúrbios do Sono recomenda que essa monitoração seja restrita a pacientes com sintomas clínicos acentuados, ou quando a polissonografia clássica não esteja disponível. Ela também é aceitável no acompanhamento terapêutico dos pacientes cujo diagnóstico já tenha sido realizado por meio da polissonografia convencional. (11) A impressão clínica isoladamente possui baixa acurácia para o diagnóstico dos distúrbios respiratórios do sono, mantendo a polissonografia imprescindível. (12,13) Em uma meta-análise de estudos clínicos para o diagnóstico de apneia obstrutiva do sono, observou-se que modelos clínicos apresentam melhores valores de OR para o diagnóstico (10,49 vs. 5,02) e para a gravidade (17,24 vs. 10,12) em comparação a modelos com o uso isolado de questionários.(14) Os elementos clínicos associados a OR > 2 foram IMC, hipertensão arterial e história de sufocamento noturno. A escala de sonolência de Epworth mostrou OR para o diagnóstico de 0,43 (IC95%: 0,13-1,48). Portanto, ainda é necessário testar modelos que possam melhorar a predição desses distúrbios, otimizando a indicação da polissonografia. O presente estudo teve como objetivo geral testar um modelo clínico de predição para o IAH, a partir de variáveis sociodemográficas e clínicas, em uma população com suspeita clínica de distúrbios do sono e encaminhada para a realização de polissonografia.
MétodosTrata-se de estudo observacional, de corte transversal. A população do estudo foi constituída por 323 pacientes consecutivos, encaminhados por médicos da rede privada de saúde a um laboratório de sono no Brasil, entre dezembro de 2006 e março de 2007, para a realização do exame de polissonografia, por suspeita clínica prévia de distúrbio do sono (roncos, apneia do sono, insônia, sonolência diurna excessiva e movimento periódico de membros inferiores durante o sono).
Os critérios de inclusão foram ter idade ≥ 18 anos e concordar em participar do estudo.
Os critérios de exclusão foram estar grávida, ter idade < 18 anos, ser portador de distúrbios psiquiátricos (história, tratamento ou uso de fármacos) ou de deficiência mental que impedisse o preenchimento dos questionários, ser analfabeto e ter estado ou estar em tratamento para apneia do sono.
O trabalho foi aprovado pelo Comitê de Ética em Pesquisa da Universidade Federal de Minas Gerais (registro 2006205212).
Utilizamos um questionário geral padronizado pela equipe clínica e adequado ao estudo, contendo variáveis sociodemográficas, clínicas e de hábitos de vida, e a escala de sonolência de Epworth (ESE).(15,16) Essa escala tem por finalidade medir a sonolência diurna subjetiva. Foi desenvolvida em 1991(15) e foi validada para uso no Brasil.(17) Utilizou-se o escore de 10 pontos como o ponto de corte na separação de níveis normais e anormais para sonolência diurna excessiva.(16)
A medida da pressão arterial foi feita com um aparelho (Missouri, Embu, Brasil) através do método indireto, com técnica auscultatória, e registrada em mmHg. Pacientes com pressão arterial ≥ 140/90 mmHg ou em uso de medicação anti-hipertensiva foram definidos como portadores de hipertensão arterial sistêmica. (18)
A altura foi medida em estadiômetro (Welmy S.A., Santa Bárbara do
Oeste, Brasil) e registrada em centímetros. A massa corpórea foi registrada em quilogramas e medida em balança (Welmy S.A.). O IMC foi calculado a partir dessas, utilizando-se a fórmula IMC = peso/altura2 (kg/m2). Pacientes com IMC de 25,0-29,9 kg/m2 foram definidos como portadores de sobrepeso, aqueles com IMC de 30,0-39,9 kg/m2 foram definidos como obesos, e aqueles com IMC > 40,0 kg/m2 foram definidos como portadores de obesidade mórbida. A circunferência do pescoço foi medida em centímetros, ao nível da articulação cricoaritenoide, utilizando-se uma fita métrica.
Realizou-se polissonografia de noite inteira com registro de 16 canais, com um mínimo de 6 horas de registro, assistida por uma profissional técnica devidamente treinada. Todos os exames foram feitos com sistemas de polissonografia (Alice 3®; Respironics, Murrysville, PA, EUA).
A frequência cardíaca de repouso foi medida por meio do sensor de eletrocardiograma do sistema de polissonografia, com um período de repouso de pelo menos 5 min.
A saturação da oxi-hemoglobina foi medida por um oxímetro digital (Healthdyne Technologies).
Os parâmetros que estabelecem a definição dos eventos, a definição das síndromes, o estabelecimento dos níveis de gravidade, a normatização dos métodos de medição e as considerações técnicas da polissonografia que foram utilizadas para a interpretação, o diagnóstico e a emissão do laudo final do exame de polissonografia seguiram as normas da American Academy of Sleep Medicine. (1)
A análise estatística foi realizada utilizando-se o programa estatístico STATA, versão 9.2 (Stata Corp., College Station, TX, EUA).
As variáveis com distribuição normal foram descritas pelas médias e desvios-padrão, enquanto aquelas com distribuição não simétrica ou desconhecida foram descritas pelas medianas e percentis 25 e 75.
A significância estatística para a comparação de variáveis categóricas foi realizada através do teste do qui-quadrado de Pearson. A comparação das médias de variáveis contínuas com distribuição normal foi feita pelo teste t de Student, e a comparação entre medianas para as variáveis com distribuição não simétrica ou desconhecida foi feita pelo teste não paramétrico de Mann-Whitney.
A regressão linear múltipla, tendo o IAH como variável dependente, foi
usada para construir um modelo de predição de apneia do sono.
Permaneceram no modelo final as variáveis que, na análise multivariada, mantiveram uma associação estatística significativa (p < 0,05). O modelo de regressão linear escolhido foi aquele que apresentou o maior coeficiente de determinação (R2) e cujas variáveis possuíam, além de uma associação estatisticamente significativa, uma associação clínica relevante com o IAH, baseada em dados da literatura.
A estatística F foi utilizada para verificar a significância estatística da inclusão no modelo de cada uma das variáveis dependentes. O coeficiente de determinação R2 foi utilizado para medir a proporção da variância total do IAH explicada por cada uma das variáveis independentes e pelo conjunto das mesmas. O coeficiente de determinação R2 ajustado foi utilizado para verificar o aumento na variância explicada no modelo multivariado, após considerar a variação no R2 devido simplesmente ao acaso.
A adequação do modelo de regressão linear foi testada graficamente, e foram utilizados os testes de pressupostos para a validade da regressão linear disponíveis no pacote estatístico STATA. Para atender a esses pressupostos (normalidade na distribuição dos resíduos, homocedasticidade e adequação do modelo no que concerne à inclusão de todas as variáveis relevantes), a variável IAH sofreu transformação logarítmica, criando-se a variável log IAH.
A normalidade na distribuição dos resíduos foi verificada graficamente e pela obtenção de um valor de p > 0,05 no teste W de Shapiro-Wilk. (19)
A hipótese de homogeneidade da variância dos resíduos (homocedasticidade) também foi verificada graficamente e pelo teste da decomposição da matriz de informação (STATA IM test) conforme proposto por Cameron & Trivedi para modelos de regressão. (20) O grau de correlação entre as variáveis incluídas no modelo foi verificado pelo fator de inflação da variância.
A adequação do modelo no que concerne à inclusão de todas as variáveis relevantes foi verificada utilizando-se o linktest e o ovtest. Outro teste utilizado foi o teste regression specification error de Ramsey.
Finalmente, foi realizada uma análise de regressão logística multinomial para verificar os fatores associados de forma independente à apneia classificada em três níveis (leve, moderada e grave) em comparação a ausência de apneia. Inicialmente, foi realizada a análise univariada para determinar a associação entre cada variável independente e a apneia do sono leve, moderada e grave. Após essa análise, foram inseridas, na análise multivariada, todas as variáveis associadas na análise inicial ao nível de p < 0,20, ficando retidas, no modelo final, apenas aquelas que apresentaram uma associação ao nível de p < 0,05. A magnitude da associação foi determinada por OR e IC95%.
ResultadosDos 323 pacientes, 191 (59,13%) eram homens, e 132 (40,87%) eram mulheres. A idade variou de 18 a 79 anos, sendo a mediana de 34,7 anos e a média de 44,6 ± 12,0 anos. Aproximadamente 75% dos pacientes situavam-se entre 30 e 60 anos de idade. Houve predomínio de casados e de pessoas com alta escolaridade (90% possuíam no mínimo o ensino médio completo, e 53,7% tinham nível superior). No que tange às medidas objetivas, 39,3% apresentaram sobrepeso; 33,1%, obesidade; e 5,6%, obesidade mórbida.
A Tabela 1 apresenta a distribuição por sexo da população estudada em relação às características investigadas. Não houve diferença entre os sexos com relação à idade e ao estado civil. Homens e mulheres diferiram estatisticamente com relação à distribuição do IMC, sendo o percentual de obesos maior em homens e o percentual de obesos mórbidos maior em mulheres. A mediana da circunferência do pescoço foi de 40 cm, com diferença significativa entre os sexos. As comorbidades mais referidas foram hipertensão arterial, refluxo gastroesofágico, rinite alérgica e depressão. As mulheres relataram mais hipotireoidismo (p < 0,001), depressão (p < 0,001) e rinite alérgica (p < 0,002) que os homens.
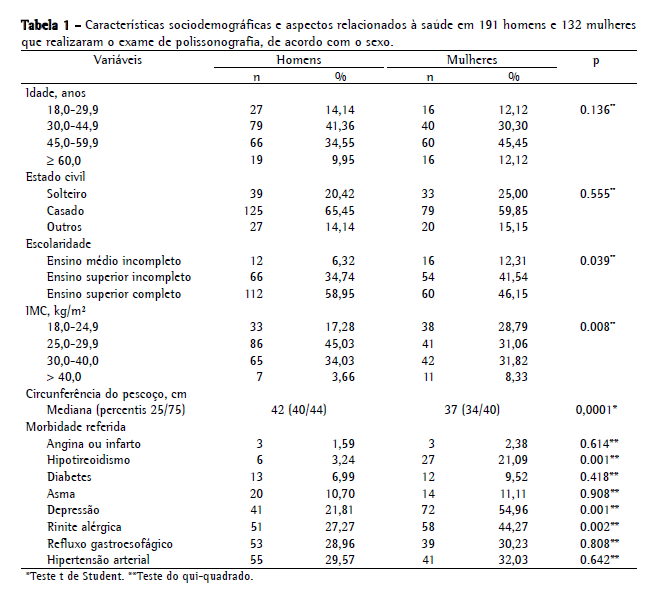
A prevalência de apneia do sono na população de estudo foi de 71,2%, sendo 30,7% classificados como de grau leve, 18,0% como de grau moderado e 22,6% como de grau grave. Os homens apresentaram apneia mais frequentemente que as mulheres (81,2% vs. 56,8%; p < 0,001) e também maior ocorrência de casos graves (30,4% vs. 11,4%). A mediana do IAH foi significativamente maior para os homens que para as mulheres (14,3 eventos/h vs. 6,2 eventos/h; p < 0,0001; Tabela 2).
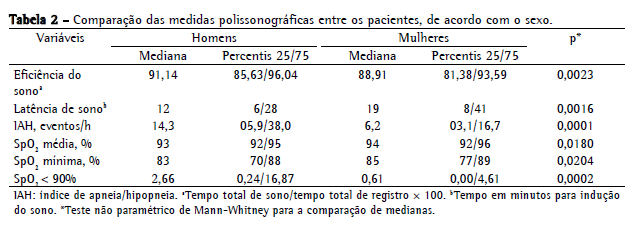
Em relação ao tipo de apneia do sono, nota-se o predomínio absoluto do tipo obstrutivo entre homens e mulheres, com os homens apresentando maior prevalência para todos os tipos de apneia (p < 0,001).
Não houve diferença significativa de idade entre os grupos com e sem apneia do sono. Nota-se, entretanto, um aumento da prevalência da doença à medida que a idade avança (60,5% entre jovens de 18-29 anos vs. 85,7% entre pacientes ≥ 60 anos). O estado civil e a escolaridade também não apresentaram diferenças significativas entre os grupos com e sem apneia do sono. Foi observada uma diferença estatisticamente significante entre o IMC e os grupos com e sem apneia do sono, com aumento da prevalência de apneia à medida que o IMC aumenta (45,1%, 73,2%, 82,2% e 94,4% para indivíduos classificados como com IMC normal, sobrepeso, obesidade e obesidade mórbida, respectivamente).
A mediana da circunferência do pescoço nos grupos sem e com apneia do sono foi de 38 cm e 42 cm, respectivamente (p < 0,001). A hipertensão arterial (p = 0,010) e o diabetes (p = 0,001) foram as comorbidades mais frequentes no grupo com apneia do sono (Tabela 3).
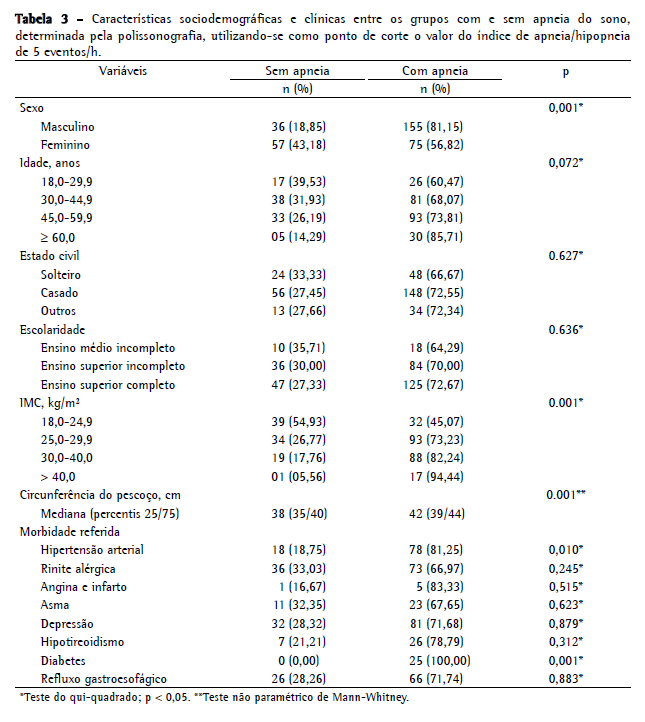
O modelo de regressão linear múltipla que melhor atendeu aos pressupostos e obteve o maior R2, tendo o log IAH como variável dependente, foi composto pelas seguintes variáveis independentes: circunferência do pescoço (cm), apneia testemunhada (sim = 1), idade (anos), IMC (kg/m2) e relato de rinite alérgica (sim = 1). Este modelo explicou aproximadamente 39% da variância do logaritmo do IAH. A equação do modelo final de regressão linear é descrita a seguir:
log IAH = −4,5552 + [apneia testemunhada × (0,5173)] + [IMC × (0,3803)] + [idade ×
(0,2604)] + [circunferência do pescoço × (0,1071)] + [rinite alérgica × (−0,2510)]
A análise de regressão logística multinomial (Tabelas 4 e 5), utilizada para investigar a associação entre as diversas variáveis independentes e a apneia do sono classificada em três níveis (leve, moderada ou grave), encontrou uma associação significativa da apneia leve com o IMC e a circunferência do pescoço. Para a apneia grave, as variáveis associadas foram idade, IMC, circunferência do pescoço e apneia testemunhada.
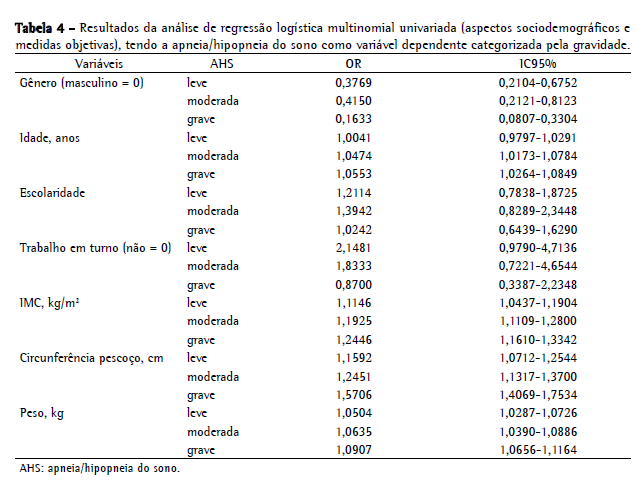
DiscussãoNo presente estudo, a apneia do sono foi diagnosticada em mais de dois terços da população estudada, sugerindo que a triagem clínica foi capaz de identificar e de excluir a maioria dos falso-positivos. A prevalência da apneia do sono no presente estudo é bem superior à relatada em estudos baseados na população geral, mas é semelhante à de relatos de estudos clínicos em populações com suspeita dessa doença. (21,22)
A frequência de apneia do sono foi significativamente superior em pacientes do sexo masculino, com uma razão de 1,43:1,00. Estudos populacionais mais recentes mostram uma razão de prevalência de 2:1 a 3:1. (23) Uma das hipóteses para justificar essa maior frequência em homens é que a apneia do sono tem apresentação clínica menos expressiva nas mulheres, o que levaria a subdiagnosticar o problema entre as mesmas, ou seja, há um provável viés de gênero. A apresentação clínica da apneia do sono é mais típica em homens, com maior frequência de roncos e apneia testemunhada. A população feminina cursa com sintomas menos específicos, tais como depressão, sonolência excessiva e fadiga, sintomas esses muitas vezes confundidos com outras causas que não a apneia do sono, o que faz com que essas pacientes sejam menos frequentemente encaminhadas à polissonografia. Estudos sobre sintomas de apneia do sono em populações clínicas mostraram que 40% das mulheres com a doença não relatavam apneia testemunhada, sufocamento noturno ou sono não reparador, enquanto que a maioria dos homens o fazia. (24)
Outros autores, estudando as diferenças da apneia do sono entre gêneros, relataram uma prevalência de 9% para mulheres e de 24% para homens, utilizando um IAH de 5 eventos/h como ponto de corte para o diagnóstico. (25)
Apesar de mais frequente em homens, o sexo não foi estatisticamente significante no modelo final de regressão linear, possivelmente devido à forte associação entre o sexo e as variáveis que permaneceram no modelo final, em especial o IMC, a circunferência do pescoço e o relato de apneia testemunhada.
A prevalência de obesidade foi elevada na população estudada, tanto a geral, medida pelo IMC, como a localizada, representada pela circunferência do pescoço. Essas duas variáveis também apresentaram relevância na predição do log IAH e associaram-se de forma independente à presença de apneia do sono para qualquer nível de gravidade, como evidenciado pelo modelo de regressão multinomial. Na população adulta de meia-idade, a obesidade é o principal fator de risco para a apneia do sono. (5,26)
A apneia do sono esteve associada com a hipertensão e o diabetes, mas não com outras comorbidades ou hábitos de vida. Em um estudo de caso-controle na população geral em que os casos de apneia do sono foram pareados a controles quanto à idade, gênero, código postal e médico consultado nos últimos 2 anos, relatou-se que os casos apresentaram maior frequência de tratamento para doenças cardiovasculares em geral, assim como para hipertensão arterial, insuficiência cardíaca congestiva, arritmia cardíaca e doença obstrutiva crônica de vias aéreas. (27) Esses achados são similares aos nossos no que concerne à hipertensão arterial. É importante lembrar que a falta de correlação dessas comorbidades no modelo final de nosso estudo pode ser justificada por falta de poder estatístico, devido ao pequeno número de casos.
Nosso modelo de regressão linear para predição do log IAH conseguiu explicar 39% da variabilidade da variável resposta. A adição de achados anatômicos das vias aéreas superiores, verificados por exame físico, tal como fora utilizado em alguns estudos prévios, mas não realizados no presente estudo, talvez elevasse o rendimento desses modelos.
Após o ajuste para as diversas variáveis de confusão na regressão logística multinomial, a idade, o IMC, a circunferência do pescoço e a apneia testemunhada permaneceram estatisticamente associadas à apneia do sono leve, moderada ou grave, sendo que o IMC foi a variável que apresentou maior força de associação para todos os níveis de gravidade.
Diversas tentativas de predição de apneia do sono sem o uso de polissonografia têm sido feitas e descritas na literatura. Esses modelos diferem entre si pela diversidade da natureza das variáveis incluídas nos estudos e pelas diferentes definições de caso, dificultando a comparação entre os resultados obtidos nesses estudos. (12,13,27,28)
O uso de análises de regressão múltipla com a inclusão de variáveis clínicas tem produzido bons modelos de predição. Quatro desses modelos de predição do IAH foram avaliados prospectivamente e mostraram ter alta sensibilidade (85-98%), mas baixa especificidade (33-39%). (29) As variáveis incluídas nesses modelos foram apneia testemunhada, hipertensão arterial, IMC, idade, roncos, circunferência do pescoço e sexo. (30)
Nosso estudo foi baseado em uma população selecionada de pacientes, o que limita a validade externa dos resultados no que concerne à estimativa de prevalência de apneia do sono. Apesar de não ser possível generalizar nossos achados para a população geral ou para uma população muito distinta da estudada no presente trabalho, a procura por modelos de predição é uma importante ferramenta para se fazer a triagem de pacientes suspeitos e maximizar a indicação da polissonografia. O uso de variáveis clínicas e sociodemográficas agregou pouco valor na diferenciação de pacientes com apneia do sono em pacientes com suspeita clínica e encaminhados para polissonografia. É possível que as informações incluídas no presente estudo expliquem melhor a variabilidade do IAH na população geral não submetida à triagem clínica.
Referências 1. Kushida CA, Littner MR, Morgenthaler T, Alessi CA, Bailey D, Coleman J Jr, et al. Practice parameters for the indications for polysomnography and related procedures: an update for 2005. Sleep. 2005;28(4):499-521.
2. Tufik S, Santos-Silva R, Taddei JA, Bittencourt LR. Obstructive sleep apnea syndrome in the Sao Paulo Epidemiologic Sleep Study. Sleep Med. 2010;11(5):441-6.
3. Bearpark H, Elliott L, Grunstein R, Cullen S, Schneider H, Althaus W, et al. Snoring and sleep apnea. A population study in Australian men. Am J Respir Crit Care Med. 1995;151(5):1459-65.
4. Peppard PE, Young T, Palta M, Dempsey J, Skatrud J. Longitudinal study of moderate weight change and sleep‑disordered breathing. JAMA. 2000;284(23):3015‑21.
5. Pack AI. Advances in sleep-disordered breathing. Am J Respir Crit Care Med. 2006;173(1):7-15.
6. Somers VK, Dyken ME, Clary MP, Abboud FM. Sympathetic neural mechanisms in obstructive sleep apnea. J Clin Invest. 1995;96(4):1897-904.
7. Row BW, Liu R, Xu W, Kheirandish L, Gozal D. Intermittent hypoxia is associated with oxidative stress and spatial learning deficits in the rat. Am J Respir Crit Care Med. 2003;167(11):1548-53.
8. O'Donnell CP, Ayuse T, King ED, Schwartz AR, Smith PL, Robotham JL. Airway obstruction during sleep increases blood pressure without arousal. J Appl Physiol. 1996;80(3):773-81.
9. Poyares D, Cintra FD, dos Santos FM, de Paola A. Complicações cardiovasculares da SAHOS: implicações e mecanismos modulatórios do sistema nervoso autônomo. In: Tufik S, editor. Medicina e biologia do sono. Barueri: Manole; 2008. p. 298-305.
10. Shahar E, Whitney CW, Redline S, Lee ET, Newman AB, Javier Nieto F, et al. Sleep-disordered breathing and cardiovascular disease: cross-sectional results of the Sleep Heart Health Study. Am J Respir Crit Care Med. 2001;163(1):19-25.
11. Togeiro SM, Smith AK. Métodos diagnósticos nos distúrbios do sono. Rev Bras Psiquiatr. 2005;27(Suppl 1):8-15.
12. Deegan PC, McNicholas WT. Predictive value of clinical features for the obstructive sleep apnoea syndrome. Eur Respir J. 1996;9(1):117-24.
13. Kushida CA, Efron B, Guilleminault C. A predictive morphometric model for the obstructive sleep apnea syndrome. Ann Intern Med. 1997;127(8 Pt 1):581-7.
14. Ramachandran SK, Josephs LA. A meta-analysis of clinical screening tests for obstructive sleep apnea. Anesthesiology. 2009;110(4):928-39.
15. Johns MW. A new method for measuring daytime sleepiness: the Epworth sleepiness scale. Sleep. 1991;14(6):540-5.
16. Johns MW. Reliability and factor analysis of the Epworth Sleepiness Scale. Sleep. 1992;15(4):376-81.
17. Bertolazi AN, Fagondes SC, Hoff LS, Pedro VD, Menna Barreto SS, Johns MW. Portuguese-language version of the Epworth sleepiness scale: validation for use in Brazil. J Bras Pneumol. 2009;35(9):877-83.
18. Sociedade Brasileira de Cardiologia [homepage on the Internet]. São Paulo: Sociedade Brasileira de Cardiologia [cited 2007 Jan 10]. V Diretrizes Brasileiras de Hipertensão Pulmonar. Available from: http://www.departamentos.cardiol.br/dha/vdiretriz/vdiretriz.asp
19. Royston JB. Some techniques for assessing multivariate based on the Shapiro-Wilk W. Appl Statist. 1983;32(2):121-33.
20. Bollen KA, Long JS, editors. Testing Structural Equation Models. Newbury Park: Sage Publications; 1993.
21. Young T, Palta M, Dempsey J, Skatrud J, Weber S, Badr S. The occurrence of sleep-disordered breathing among middle-aged adults. N Engl J Med. 1993;328(17):1230-5.
22. Daltro CH, Fontes FH, Santos-Jesus R, Gregorio PB, Araújo LM. Obstructive sleep apnea and hypopnea syndrome (OSAHS): association with obesity, gender and age [Article in Portuguese]. Arq Bras Endocrinol Metabol. 2006;50(1):74-81.
23. Kapsimalis F, Kryger MH. Gender and obstructive sleep apnea syndrome, part 1: clinical features. Sleep. 2002;25(4):409-16.
24. Ambrogetti A, Olson LG, Saunders NA. Differences in the symptoms of men and women with obstructive sleep apnoea. Aust N Z J Med. 1991;21(6):863-6.
25. Lin CM, Davidson TM, Ancoli-Israel S. Gender differences in obstructive sleep apnea and treatment implications. Sleep Med Rev. 2008;12(6):481-96.
26. Banno K, Kryger MH. Sleep apnea: clinical investigations in humans. Sleep Med. 2007;8(4):400-26.
27. Smith R, Ronald J, Delaive K, Walld R, Manfreda J, Kryger MH. What are obstructive sleep apnea patients being treated for prior to this diagnosis? Chest. 2002;121(1):164-72.
28. Crocker BD, Olson LG, Saunders NA, Hensley MJ, McKeon JL, Allen KM, et al. Estimation of the probability of disturbed breathing during sleep before a sleep study. Am Rev Respir Dis. 1990;142(1):14-8.
29. Dixon JB, Schachter LM, O'Brien PE. Predicting sleep apnea and excessive day sleepiness in the severely obese: indicators for polysomnography. Chest. 2003;123(4):1134-41.
30. Rowley JA, Aboussouan LS, Badr MS. The use of clinical prediction formulas in the evaluation of obstructive sleep apnea. Sleep. 2000;23(7):929-38.
* Trabalho realizado na Faculdade de Medicina da Universidade Federal de Minas Gerais, Belo Horizonte (MG) Brasil.
Endereço para correspondência: Silvio Musman. Rua Padre Rolim, 375, Santa Efigênia, CEP 30130-090, Belo Horizonte, MG, Brasil.
Tel 55 31 3222-6004. E-mail: silviomusman@yahoo.com.br
Apoio financeiro: Sandhi Maria Barreto e Valéria Maria de Azeredo Passos são bolsistas de produtividade em pesquisa do Conselho Nacional de Desenvolvimento Científico e Tecnológico (CNPq; processos nº 00908/95 e nº 300159/99-4). Izabella Barreto Romualdo Silva foi bolsista do Programa Institucional de Bolsas de Iniciação Científica (PIBIC) do CNPq.
Recebido para publicação em 6/4/2010. Aprovado, após revisão, em 3/9/2010.
Sobre os autoresSilvio Musman
Médico Pneumologista. Hospital Júlia Kubitschek, Fundação Hospitalar do Estado de Minas Gerais - FHEMIG - Belo Horizonte (MG) Brasil.
Valéria Maria de Azeredo Passos
Professora Associada. Departamento de Clínica Médica, Faculdade de Medicina, Universidade Federal de Minas Gerais, Belo Horizonte (MG) Brasil.
Izabella Barreto Romualdo Silva
Graduanda em Medicina. Faculdade de Medicina, Universidade Federal de Minas Gerais, Belo Horizonte (MG) Brasil.
Sandhi Maria Barreto
Professora Adjunta. Faculdade de Medicina, Universidade Federal de Minas Gerais, Belo Horizonte (MG) Brasil. Consultora. Grupo Técnico Consultor em Doenças e Agravos Não Transmissíveis, Coordenação de Doenças e Agravos Não Transmissíveis, Departamento de Análise de Saúde, Secretaria de Vigilância em Saúde, Ministério da Saúde, Brasília (DF) Brasil.