ABSTRACT
Objective: To evaluate autonomic modulation in individuals with COPD, compared with healthy controls, via recurrence plots (RPs) and linear heart rate variability (HRV) indices. Methods: We analyzed data on 74 volunteers, who were divided into two groups: COPD (n = 43) and control (n = 31). For calculation of HRV indices, heart rate was measured beat-by-beat during 30 min of supine rest using a heart-rate meter. We analyzed linear indices in the time and frequency domains, as well as indices derived from the RPs. Results: In comparison with the control group, the COPD group showed significant increases in the indices derived from the RPs, as well as significant reductions in the linear indices in the time and frequency domains. No significant differences were observed in the linear indices in the frequency domains expressed in normalized units or in the low frequency/high frequency ratio. Conclusions: Individuals with COPD show a reduction in both sympathetic and parasympathetic activity, associated with decreased complexity of autonomic nervous system function, as identified by RPs, which provide important complementary information in the detection of autonomic changes in this population.
Keywords:
Pulmonary disease, chronic obstructive; Autonomic nervous system; Nonlinear dynamics; Recurrence; Heart rate; Sympathetic nervous system.
RESUMO
Objetivo: Avaliar a modulação autonômica de indivíduos com DPOC, comparados com um grupo controle saudável, por meio do plot de recorrência (PR) associado a métodos lineares da variabilidade da frequência cardíaca (VFC). Métodos: Foram avaliados dados de 74 voluntários, os quais foram divididos em grupos DPOC (n = 43) e controle (n = 31). Para a análise dos índices de VFC, a frequência cardíaca dos voluntários foi registrada, batimento a batimento, por meio de um cardiofrequencímetro com os voluntários em repouso na posição supina por 30 min. Foram avaliados índices lineares nos domínios de tempo e de frequência, assim como índices obtidos por meio do PR. Resultados: No grupo DPOC, em comparação com o grupo controle, houve aumentos significativos em índices obtidos do PR, assim como houve reduções significativas nos índices lineares nos domínios de tempo e de frequência. Não foram observadas diferenças significativas nos índices lineares nos domínios de frequência em unidades normalizadas e na relação entre os componentes de alta e baixa frequência. Conclusões: Indivíduos com DPOC apresentam uma diminuição tanto da atividade simpática quanto da parassimpática, associada a uma redução da complexidade do sistema nervoso autônomo, identificada pelo PR, que fornece informações complementares importantes na detecção de alterações autonômicas nessa população.
Palavras-chave:
Doença pulmonar obstrutiva crônica; Sistema nervoso autônomo; dinâmica não linear; Recidiva; Frequência cardíaca, Sistema nervoso simpático.
INTRODUÇÃOA DPOC, caracterizada pela obstrução ou limitação crônica do fluxo aéreo não inteiramente reversível,(1) atinge três milhões de indivíduos no mundo,(2) ocupa o quarto lugar entre as causas mundiais de óbitos(3) e está associada a inúmeras complicações, dentre as quais se destacam as alterações no sistema nervoso autônomo (SNA).(4,5)
O SNA é um exemplo de sistema com dinâmica não linear(6) que tem influência na frequência cardíaca e na pressão arterial a fim de garantir um adequado funcionamento dos órgãos do corpo para que suas reais necessidades sejam supridas. Esse sistema pode ser avaliado pela variabilidade da frequência cardíaca (VFC) e reflete ao longo do tempo a capacidade do coração de reagir frente às mudanças autonômicas.(7)
Estudos que avaliam o SNA na DPOC por meio da VFC utilizam principalmente índices lineares nos domínios do tempo e da frequência para essa análise e apontam que esses pacientes apresentam uma diminuição desses índices em condições de repouso quando comparados a sujeitos controles da mesma faixa etária.(8) Os poucos estudos que utilizaram métodos não lineares nessa população demonstraram uma redução das propriedades de correlação fractal de curto prazo da frequência cardíaca(9) e uma menor dispersão dos intervalos RR de um eletrocardiograma, batimento a batimento, expressas pela análise visual do plot de Poincaré,(4) indicando uma menor VFC nos indivíduos com DPOC.
As análises da VFC por métodos não lineares vêm ganhando crescente interesse, pois há evidências de que os mecanismos que envolvem a regulação cardiovascular provavelmente interagem entre si de maneira não linear. (10) Um dos métodos utilizados para esse fim é o plot de recorrência (PR), inicialmente proposto por Eckmann et al.(11) como uma ferramenta gráfica para o diagnóstico de flutuações e periodicidades ocultas na evolução temporal, as quais não são notadas por outros métodos,(12) pelo qual se obtêm medidas baseadas, principalmente, em linhas diagonais orientadas na trama da reincidência, como a recurrence rate (REC, taxa de recorrência), o determinismo (DET) e a entropia, entre outras.(12,13)
Os métodos não lineares tradicionais são limitados a sinais estacionários longos, uma condição raramente conhecida na biologia,(14) enquanto o PR foi desenvolvido para localizar mudanças não estacionárias e estruturais,(11) podendo ser uma ferramenta mais sensível para se detectar alterações fisiológicas(15) e contribuir na vigilância e no monitoramento de indivíduos com a doença.
Diante do exposto, pretende-se, com o presente estudo, avaliar a modulação autonômica em pacientes com DPOC e em indivíduos sem a doença, por meio da análise dos índices do PR, juntamente com índices de VFC nos domínios do tempo e da frequência. Hipotetizamos que pacientes com DPOC apresentarão uma redução da modulação autonômica e que o PR se mostrará uma ferramenta sensível para identificar essa condição.
MÉTODOSPopulação de estudoEste é um estudo prospectivo de caso controle. Para a realização do presente estudo, foram recrutados 74 voluntários, de ambos os sexos, os quais foram divididos em dois grupos: DPOC (n = 43) e controle (n = 31), levando em consideração os critérios estabelecidos pelo Global Initiative for Chronic Obstructive Lung Disease (GOLD).(1)
No grupo DPOC foram incluídos indivíduos ex-tabagistas com diagnóstico médico de DPOC, confirmado pelo teste de função pulmonar com padrão obstrutivo, curvas reprodutíveis e sem exacerbações do quadro de DPOC nos últimos dois meses. Já para o grupo controle foram incluídos aqueles com a mesma faixa etária, não tabagistas, e que não apresentassem diagnóstico de DPOC, confirmado pelo teste de função pulmonar com padrão normal e curvas reprodutíveis.
Para ambos os grupos não foram incluídos indivíduos com pelo menos uma das seguintes características: alcoólatras, aqueles em uso de medicação que influenciassem a modulação autonômica e aqueles que apresentassem doenças cardíacas e/ou metabólicas.
Os procedimentos do estudo foram aprovados pelo Comitê de Ética em pesquisa da Faculdade de Ciências e Tecnologia, Universidade Estadual Paulista (CAAE no. 15922813.9.0000.5402-306.419), localizada na cidade de Presidente Prudente (SP), e todos os voluntários foram devidamente informados sobre os procedimentos e objetivos do estudo. Após concordarem em participar do estudo, assinaram um termo de consentimento livre e esclarecido.
Protocolo experimentalO protocolo experimental foi composto por duas etapas. A primeira consistiu em uma avaliação inicial com o objetivo de obter informações quanto à identificação do voluntário e de avaliar tanto as medidas antropométricas quanto a função pulmonar. Na segunda etapa, realizada após 24 h, os voluntários tiveram sua frequência cardíaca registrada, batimento a batimento, durante 30 min por meio de um cardiofrequencímetro (Polar S810i; Polar Electro, Kempele, Finlândia) para a posterior análise dos índices de VFC.
Avaliação inicialOs voluntários foram identificados quanto a seu nome, idade e uso de medicamentos e tiveram avaliadas as medidas antropométricas (massa corpórea e altura) e a função pulmonar. A massa corpórea foi obtida por meio de uma balança digital eletrônica (Lumina MEA-02550; Plenna, São Paulo, Brasil), e a altura foi mensurada por meio de um estadiômetro (Personal Caprice; Sanny, São Bernardo do Campo, Brasil) com o voluntário em posição ereta e sem sapatos. A partir dos dados obtidos, foi calculado o índice de massa corpórea (IMC) pela seguinte fórmula: IMC = peso/altura2 (kg/m2).(16)
Para a análise da função pulmonar, os voluntários foram submetidos ao teste de espirometria utilizando o espirômetro Spirobank (MIR, Roma, Itália).(17,18) Foram obtidas três curvas aceitáveis e duas reprodutíveis, com um limite máximo de oito tentativas. O distúrbio obstrutivo foi identificado conforme as diretrizes da GOLD.(1)
Avaliação da modulação autonômicaApós 24 h da primeira etapa, os voluntários realizaram a avaliação da modulação autonômica. Para essa avaliação eles foram orientados a não consumirem bebidas alcoólicas e/ou estimulantes do SNA, como café, chá, refrigerantes e achocolatados, assim como não realizarem exercícios físicos intensos durante as 24 h precedentes ao teste.
Para a avaliação da modulação autonômica, a frequência cardíaca foi captada batimento a batimento no período da manhã (de 8 h a 11 h) em um ambiente tranquilo, com temperatura ambiente entre 21°C e 24°C e umidade relativa do ar de 40% a 60%.
Para essa captação, foi posicionada, no terço distal do esterno, uma cinta de captação e, no punho, um cardiofrequencímetro (Polar Electro), equipamento previamente validado para a captação da frequência cardíaca batimento a batimento e para sua utilização para o cálculo dos índices de VFC.(10) Os voluntários foram instruídos a manter-se em silêncio, acordados, em repouso, com respiração espontânea por 30 min em decúbito dorsal em uma maca. Após essa avaliação os voluntários foram liberados.
Para a análise dos índices de VFC, foram utilizados 1.000 intervalos RR obtidos do trecho mais estável do traçado. A série de intervalos RR passou inicialmente por uma filtragem, utilizando-se o filtro padrão do software Polar ProTrainer 5, versão 5.41.002 (Polar Electro),(19) usando um filtro moderado (zona de proteção mínima de seis batimentos), e, posteriormente, foi realizada uma inspeção visual na série temporal de intervalos RR no monitor do computador, a qual mostrou ausência de artefatos que pudessem interferir nas análises de VFC. Somente séries que apresentaram mais de 95% de batimentos sinusais foram incluídas no estudo.(10)
Os cálculos dos índices de VFC no domínio do tempo e da frequência foram feitos por meio do software Kubios HRV, versão 2.0 (Kubios Oy; Kuopio, Finlândia), enquanto os índices do PR foram calculados no Visual Recurrence Analyses, versão 4.9 (Eugene Kononov, Springfield, MA, EUA).
Foram analisados, no domínio do tempo, os índices standard deviation of the NN interval (SDNN, desvio-padrão dos intervalos NN), representando todos os intervalos RR normais, e root mean square of the successive differences (RMSSD, raiz quadrada das diferenças sucessivas), que corresponde à raiz quadrada da somatória do quadrado das diferenças entre os intervalos RR no registro, dividido pelo número de intervalos RR em um tempo determinado menos um intervalo RR.(10)
No domínio da frequência, foram utilizados os componentes espectrais de low frequency (LF; baixa frequência; 0,04-0,15 Hz) e de high frequency (HF; alta frequência; 0,15-0,40 Hz), em ms² e em unidades normalizadas (un), assim como a razão entre esses componentes (LF/HF). A análise espectral foi calculada utilizando o algoritmo da transformada rápida de Fourier.(10)
A análise do PR foi feita de forma qualitativa e quantitativa. A análise qualitativa foi realizada pela visualização do plot, e a quantitativa a partir dos seguintes índices: REC, DET, entropia de Shannon (ES), laminaridade (LAM), trapping time (TT) e linha máxima (MaxLine). Os seguintes parâmetros foram utilizados no PR: dimensão de imersão (embedding dimension) = 10; tempo de retardo (time delay) = 1, raio = 70, linha = 2(20) e esquema de cores em cinza.
O PR visualiza o comportamento de trajetórias no espaço de fase e mostra todos os tempos no qual um sistema dinâmico se repete.(11) O gráfico do PR é definido como sendo uma matriz simétrica composta de zeros e uns, sendo que PR(i,j) é 1 se o vetor ξi sobre a trajetória é fechado para o vetor ξj.(21)
onde d(ξi − ξj) é a distância euclidiana, r é um limiar fixo, e cc significa "caso contrário".
A partir dessa matriz calculam-se vetores, por meio da reconstrução do espaço, e a soma desses vetores possibilita encontrar valores das distâncias euclidianas. Os valores dessas distâncias são comparados ao valor de r, que possibilita a construção de um gráfico. Se a distância entre os dois vetores ξi e ξj sobre a trajetória reconstruída for menor do que r, um ponto preto representa a posição (i,j) da matriz; caso contrário, a posição é representada por um ponto branco.(22)
A REC representa a probabilidade de estados similares ocorrerem dentro de um mesmo sistema.(23) O DET representa a proporção de pontos recorrentes que formam linhas diagonais, pelas quais sistemas com espaços de fase similares ou iguais permanecem nas mesmas regiões por um determinado tempo.(23) A LAM é representada pelo raio entre os pontos de recorrência que formam linhas verticais, determinando a ocorrência de estados laminares em um sistema.(24) A quantidade e o comprimento das estruturas verticais são definidos como TT.(24) A MaxLine é definida como o comprimento máximo das estruturas diagonais no PR.(25) E, finalmente, obtemos a entropia, definida pela distribuição de frequência de acordo com a distribuição das linhas diagonais.(15) Se refere à ES na probabilidade p(l) = P(l)/Nl de se encontrar a linha diagonal do comprimento l (Nl = Σl ≥ l min P (l)), onde l é o número de linhas diagonais, Ni é o número total de linhas diagonais, e P é a probabilidade.
Análise dos dadosPara a caracterização da amostra foi utilizada a estatística descritiva, e os resultados foram apresentados com valores de média, mediana, mínimo e máximo. Para a comparação das variáveis antropométricas, idade, valores espirométricos e índices da VFC entre os grupos, inicialmente foi testada a normalidade dos dados por meio do teste de Shapiro-Wilk; se aceita a distribuição normal, foi aplicado o teste t de Student para dados não pareados (idade, peso, altura, CVF, VEF1/CVF, REC, TT, ES, SDNN, LF/HF), enquanto, para as distribuições não normais, foi aplicado o teste de Mann-Whitney (IMC, VEF1, DET, LAM, MaxLine, RMSSD, LF [ms2], HF [ms2], LF [un] e HF [un]). Foram consideradas diferenças estatisticamente significantes os valores de p menores que 0,05.
RESULTADOSA Tabela 1 apresenta as características dos voluntários dos dois grupos estudados. Diferenças significantes entre os grupos foram observadas para altura, CVF, VEF1 e VEF1/CVF.
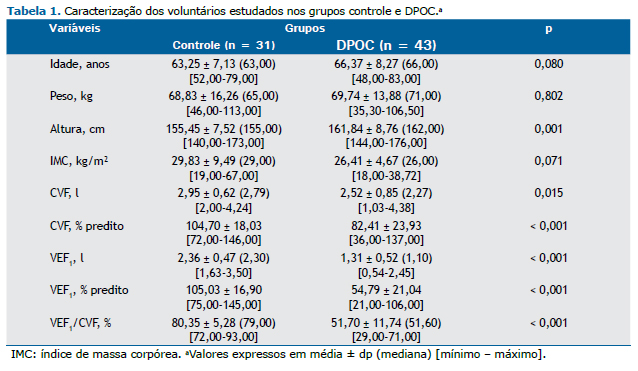
Os índices lineares da VFC analisados nos domínios do tempo e da frequência estão apresentados na Tabela 2. Menores valores dos índices SDNN, RMSSD, LF(ms2) e HF (ms2) foram observados no grupo DPOC em comparação ao grupo controle (p < 0,05). Não foram encontradas diferenças estatisticamente significantes entre os grupos para os índices LF (un), HF (un) e relação LF/HF.
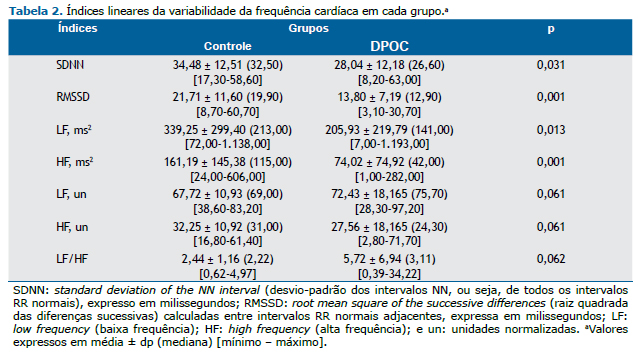
Na Tabela 3 estão apresentados os índices calculados por meio do PR dos grupos estudados. Aumentos de todos os índices obtidos pelo PR foram observados para o grupo DPOC (p < 0,05).
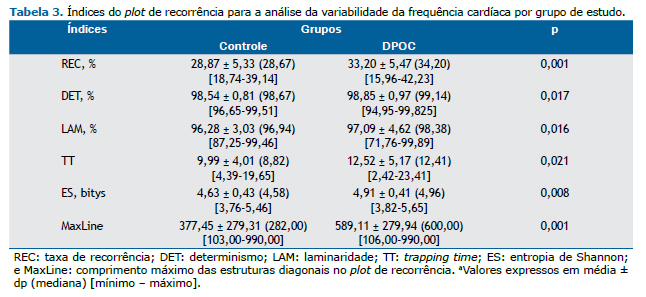
A Figura 1 mostra um exemplo representativo da análise visual do PR. Observa-se a presença de mais pontos de mesmo estado de configuração (pontos pretos) no grupo DPOC em comparação à no grupo controle.
DISCUSSÃOOs resultados do presente estudo sugerem que indivíduos com DPOC apresentam perda da complexidade do SNA associada a reduções tanto da atividade simpática quanto da parassimpática. Ainda, houve aumentos de todos os índices derivados do PR no grupo DPOC quando comparados com o grupo controle.
Os índices REC e DET relacionam-se com a complexidade da modulação autonômica cardíaca. (21) Segundo Webber et al.,(15) altos valores de REC são encontrados em sistemas periódicos quando comparados a sistemas não periódicos, e sistemas estruturados e determinísticos apresentam elevadas taxas de DET. Considerando que sistemas periódicos e estruturados são menos complexos, altos valores de REC e DET indicam uma menor complexidade da modulação autonômica, o que pode ser observado nos pacientes com DPOC analisados no presente estudo.
Segundo Javorka et al.,(6) quanto menores os valores dos índices TT e LAM, maior é a complexidade de um sistema dinâmico. Nos pacientes com DPOC, observamos aumento desses índices em relação aos indivíduos controle, sugerindo novamente menor complexidade nesses indivíduos.
Em relação a ES, baixos valores parecem estar associados à estocasticidade enquanto elevados valores se relacionam a um comportamento mais determinístico. (21) No presente estudo, observamos que o grupo DPOC apresentou maiores valores de ES, o que indica um sistema mais determinístico e, portanto, menor complexidade do SNA nesses indivíduos.
Finalmente, foram observados maiores valores de MaxLine nos indivíduos com DPOC em comparação aos do grupo controle. No gráfico, esse índice representa um maior comprimento das linhas diagonais,(25) e sabe-se que essas correspondem à persistência do estado durante um intervalo de tempo(22); portanto, quanto maior for o valor desse índice, menos caótico é considerado o sistema,(26) o que novamente retrata uma menor complexidade da modulação autonômica no grupo DPOC.
A análise qualitativa realizada pela visualização do PR também demonstra que indivíduos com DPOC apresentam uma menor complexidade do SNA quando comparados com indivíduos saudáveis. Em indivíduos com DPOC, nota-se a presença de mais pontos de mesmo estado de configuração (pretos), diferentemente do encontrado em indivíduos sem a doença, onde é possível observar a presença de um maior número de pontos com um diferente estado de configuração (brancos).
A análise das linhas diagonais, horizontais e verticais presentes no PR nos possibilita uma rápida interpretação visual das alterações na modulação autonômica de indivíduos em condições patológicas. (21) Segundo Assmann et al.,(22) as linhas diagonais indicam uma semelhante evolução entre as diferentes partes do trajeto, enquanto as linhas horizontais e verticais mostram que o sistema não muda por algum tempo. (22) Como relatado por Ferreira,(21) para séries temporais de sujeitos saudáveis, o PR tem uma linha diagonal e menos quadrados aparentes, o que indica uma VFC mais alta. Com isso, podemos observar maiores pontos recorrentes na Figura 1A, quando comparada com os da Figura 1B, indicando um sistema mais recorrente e menos dinâmico no grupo DPOC e uma menor complexidade da modulação autonômica nessa população.
A identificação de alterações autonômicas por meio de índices não lineares da VFC tem se destacado na literatura em função de evidências que indicam que os mecanismos envolvidos na regulação cardiovascular interagem entre si de forma não linear,(10) permitindo um melhor entendimento dos sistemas complexos e dinâmicos do corpo humano,(27) o que fornece informações adicionais relacionadas à interpretação fisiológica e ao prognóstico dos indivíduos avaliados.(28) Nesse sentido, Carvalho et al.(9) estudaram a dinâmica fractal da frequência cardíaca em sujeitos com e sem DPOC por meio da mensuração de expoentes fractais de curto e longo prazo e relataram uma diminuição das propriedades de correlação fractal de curto prazo das frequências cardíacas no grupo DPOC, indicando uma redução na complexidade autonômica desses indivíduos, como também observado em nosso estudo pelo PR.
A análise por meio da dinâmica fractal difere em alguns aspectos da análise do PR. A dinâmica fractal foi desenvolvida para caracterizar flutuações em escalas, ou seja, séries temporais de curta e de longa duração,(20) enquanto o PR foi desenvolvido para localizar mudanças não estacionárias e estruturais,(11) permitindo, dessa forma, a identificação de flutuações e periodicidades ocultas na evolução temporal.(12) Assim, a utilização do PR como ferramenta de análise da VFC pode fornecer informações complementares importantes na detecção de alterações autonômicas de indivíduos com DPOC.
O estado saudável de um indivíduo é caracterizado por certo grau de caoticidade do SNA, e anormalidades da função desse sistema provocam a diminuição do caos cardíaco.(21,29) Alterações na modulação autonômica podem determinar uma marcada redução da complexidade da dinâmica das flutuações de frequência cardíaca, produzindo uma menor adaptação do ritmo cardíaco e uma menor capacidade do mesmo para enfrentar as demandas de um ambiente continuamente em mudanças.(25,30)
Tomados em conjunto, esses dados sugerem que pacientes com DPOC apresentam uma diminuição da complexidade da modulação autonômica e, consequentemente, estão sujeitos a uma pior condição de saúde. A perda da complexidade da modulação autonômica cardíaca está associada a eventos clínicos adversos, como doença arterial coronariana com estenose ≥ 50%,(31) diabetes mellitus tipo 1(6) e esquizofrenia,(32) bem com o processo de envelhecimento.(33)
Associada à perda da complexidade do SNA, observa-se, em nossos achados, a redução dos índices LF e HF em ms2, bem como dos índices SDNN e RMSSD, o que sugere uma diminuição tanto da variabilidade global como da atividade parassimpática e simpática.
Carvalho et al.,(9) em seus achados, também encontraram reduções desses índices ao comparar idosos portadores de DPOC com sujeitos controle da mesma faixa etária. Índices geométricos também apontaram para uma diminuição da atividade vagal (desvio-padrão 1) e da variabilidade global (desvio-padrão 2, interpolação triangular do histograma do intervalo NN e índice triangular) em pacientes com DPOC.(4) Diversos outros estudos corroboram esses achados, que apontam para uma alteração na modulação autonômica cardíaca, demonstrando um prejuízo dessa atividade nos indivíduos com DPOC.(34-38)
Além disso, Mazzuco et al.(39) identificaram que, quanto maior for o comprometimento da função pulmonar, menor é a dinâmica da frequência cardíaca no indivíduo com DPOC, resultado esse evidenciado por índices lineares e não lineares da VFC. Ainda, segundo os mesmos autores, existe nesses indivíduos uma relação negativa entre a DLCO e os intervalos RR durante o estímulo parassimpático (manobra de arritmia sinusal respiratória), o que pode estar relacionado a uma maior estimulação simpática que altera o tônus capilar pulmonar.
Ainda não está bem estabelecido o mecanismo pelo qual a modulação autonômica está alterada na DPOC. Hipóteses são consideradas em relação ao tônus predominante nesses casos, pois a hiperinsuflação característica do quadro poderia gerar impulsos vagais alterados.(40) Sendo assim, os índices lineares de VFC apontam que indivíduos com DPOC apresentam uma redução tanto da atividade simpática quanto da parassimpática, e as análises do PR mostram uma perda da complexidade autonômica, indicando piores condições de saúde nessa população. Além disso, os resultados contribuem com novas informações à literatura no que diz respeito a um novo método eficaz que pode detectar alterações relacionadas ao SNA em indivíduos com DPOC por meio da localização de alterações não estacionárias e estruturais.
Pode-se citar como uma limitação do presente estudo a heterogeneidade do grau de obstrução dos pacientes inseridos no grupo DPOC. O grupo foi composto por 3 pacientes classificados como GOLD I (VEF1 ≥ 80% do previsto), 18 pacientes classificados como GOLD II (50% < VEF1 < 80% do previsto), 17 classificados como GOLD III (30% < VEF1 < 50% do previsto), e 5 pacientes classificados como GOLD IV (VEF1 < 30% do previsto). Apesar de esse ser um grupo heterogêneo quanto ao grau de gravidade da doença, Camilo et al.,(5) em seus achados, afirmam que esse aspecto não afeta de forma significativa as análises da VFC. Ainda a respeito das limitações, podemos mencionar o uso de medicação broncodilatadora. Porém, em nosso estudo, os sujeitos não estavam sob o efeito diário dessa medicação durante o período de coleta de dados, e, como mencionado anteriormente, nenhum deles apresentou exacerbações recentes da DPOC, fato que pode suprir tal limitação.
Conclui-se, portanto, que indivíduos com DPOC apresentam uma diminuição da complexidade do SNA, demonstrada pelos índices do PR, associada à redução tanto da atividade simpática quanto da parassimpática, observada pelos índices lineares da VFC.
REFERÊNCIAS1. Global Initiative for Chronic Obstructive Lung Disease. Global strategy for the diagnosis, management, and prevention of chronic obstructive pulmonary disease. Updated 2010. Bethesda: Global Initiative for Chronic Obstructive Lung Disease; 2011.
2. World Health Organization (WHO). Prevention and Control of Noncommunicable Diseases: Guidelines for Primary Health Care in low-resource settings. Geneva: WHO; 2012.
3. Wehrmeister FC, Knorst M, Jardim JR, Macedo EC, Noal RB, Martínez-Mesa J, et al. Pulmonary rehabilitation programs for patients with COPD. J Bras Pneumol. 2011;37(4):544-55. https://doi.org/10.1590/S1806-37132011000400017
4. Carvalho TD, Pastre CM, Rossi RC, Abreu LC, Valenti VE, Vanderlei LC. Geometric index of heart rate variability in chronic obstructive pulmonary disease. Rev Port Pneumol. 2011;17(6):260-5.
5. Camillo CA, Laburu Vde M, Gonçalves NS, Cavalheri V, Tomasi FP, Hernandes NA, et al. Improvement of heart rate variability after exercise training and its predictors in COPD. Respir Med. 2011;105(7):1054-62. https://doi.org/10.1016/j.rmed.2011.01.014
6. Javorka M, Trunkvalterova Z, Tonhajzerova I, Lazarova Z, Javorkova J, Javorka K. Recurrences in heart rate dynamics are changed in patients with diabetes mellitus. Clin Physiol Funct Imaging. 2008;28(5):326-31. https://doi.org/10.1111/j.1475-097X.2008.00813.x
7. Stuckey MI, Tulppo MP, Kiviniemi AM, Petrella RJ. Heart rate variability and the metabolic syndrome: a systematic review of the literature. Diabetes Metab Res Rev. 2014;30(8):784-93 https://doi.org/10.1002/dmrr.2555
8. Pantoni CB, Reis MS, Martins LE, Catai AM, Costa D, Borghi-Silva A. Study on autonomic heart rate modulation at rest among elderly patients with chronic obstructive pulmonary disease. Rev Bras Fisioter. 2007;11(1):35-41.
9. Carvalho TD, Pastre CM, de Godoy MF, Fereira C, Pitta FO, de Abreu LC, et al. Fractal correlation property of heart rate variability in chronic obstructive pulmonary disease. Int J Chron Obstruct Pulmon Dis. 2011;6:23-8. https://doi.org/10.2147/COPD.S15099
10. Vanderlei LC, Pastre CM, Hoshi RA, Carvalho TD, Godoy MF. Basic notions of heart rate variability and its clinical applicability. Rev Bras Cir Cardiovasc. 2009;24(2):205-17. https://doi.org/10.1590/S0102-76382009000200018
11. Eckmann JP, Kamphorst SO, Ruelle D. Recurrence Plots of Dynamical Systems. Europhys Lett. 1987;4(9):973-7. https://doi.org/10.1209/0295-5075/4/9/004
12. Wessel N, Marwan N, Meyerfeldt U, Schirdewan A, Kurths J. Recurrence Quantification Analysis to Characterise the Heart Rate Variability Before the Onset of Ventricular Tachycardia. In: Crespo J, Maojo V, Martin F. (editors) Medical Data Analysis. ISMDA 2001. Lecture Notes in Computer Science, vol 2199. Berlin, Heidelberg: Springer; 2001. https://doi.org/10.1007/3-540-45497-7_45
13. Marwan N, Romano MC, Thiel M, Kurths J. Recurrence plots for the analysis of complex systems. Phys Rep. 2007;438(5-6):237-329. https://doi.org/10.1016/j.physrep.2006.11.001
14. Schreiber T. Interdisciplinary application of nonlinear time series methods. Phys Rep. 1999;308(1):1-64. https://doi.org/10.1016/S0370-1573(98)00035-0
15. Webber CL Jr, Zbilut JP. Dynamical assessment of physiological systems and states using recurrence plot strategies. J Appl Physiol (1985). 1994;76(2):965-73.
16. Associação Brasileira para o Estudo da Obesidade e da Síndrome Metabólica (ABESO) [homepage on the Internet]. São Paulo: ABESO [cited 2017 Mar 1]. Diretrizes Brasileiras de Obesidade 2009-2010. 3rd Ed. [Adobe Acrobat document, 85p.]. Available from: http://www.abeso.org.br/pdf/diretrizes_brasileiras_obesidade_2009_2010_1.pdf
17. Sociedade Brasileira de Pneumologia e Tisiologia. Diretrizes para teste de função pulmonar. J Pneumol. 2002;28(Suppl 3):S51-S238.
18. Miller MR, Hankinson J, Brusasco V, Burgos F, Casaburi R, Coates A, et al. Standardisation of spirometry. Eur Respir J. 2005;26(2):319-38. https://doi.org/10.1183/09031936.05.00034805
19. Kiviniemi AM, Hautala AJ, Kinnunen H, Tulppo MP. Endurance training guided individually by daily heart rate variability measurements. Eur J Appl Physiol. 2007;101(6):743-51. https://doi.org/10.1007/s00421-007-0552-2
20. Baptista MA. Gráficos de recorrência e de poincaré na análise da quantidade de internações por diferentes grupos nosológicos, ocorridas ao longo de uma década, em um hospital de ensino [thesis]. São José do Rio Preto: Faculdade de Medicina de São José do Rio Preto; 2011.
21. Ferreira MT. Métodos lineares e não lineares de análise de séries temporais e sua aplicação no estudo da variabilidade da frequência cardíaca de jovens saudáveis [dissertation]. Botucatu: Universidade Estadual Paulista; 2010. Available from: http://base.repositorio.unesp.br/handle/unesp/95057
22. Assmann B, Thiel M, Romano MC, Niemitz C. Recurrence plot analyses suggest a novel reference system involved in newborn spontaneous movements. Behav Res Methods. 2006;38(3):400-6. https://doi.org/10.3758/BF03192793
23. Marwan N, Kurths J. Nonlinear analysis of bivariate data with cross recurrence plots. Phys Lett A. 2002;302(5-6):299-307. https://doi.org/10.1016/S0375-9601(02)01170-2
24. Marwan N, Wessel N, Meyerfeldt U, Schirdewan A, Kurths J. Recurrence-plot-based measures of complexity and their application to heart-rate-variability data. Phys Rev E Stat Nonlin Soft Matter Phys. 2002;66(2 Pt 2):026702. https://doi.org/10.1103/PhysRevE.66.026702
25. Marwan N, Donges JF, Zou Y, Donner RV. Complex network approach for recurrence analysis of time series. Phys Lett A. 2009;373(46):4246-54. http://dx.doi.org/10.1016/j.physleta.2009.09.042 https://doi.org/10.1016/j.physleta.2009.09.042
26. Trulla LL, Giuliani A, Zbilut JP, Webber CL. Recurrence quantification analysis of the logistic equation with transients. Phys Lett A. 1996;223(4):255-60. https://doi.org/10.1016/S0375-9601(96)00741-4
27. Higgins JP. Nonlinear systems in medicine. Yale J Biol Med. 2002;75(5-6):247-60.
28. Khaled AS, Owis MI, Mohamed AS. Employing time-domain methods and poincaré plot of heart rate variability signals to detect congestive heart failure. BIME J. 2006;6(1):35-41.
29. Wagner CD, Persson PB. Chaos in the cardiovascular system: an update. Cardiovasc Res. 1998;40(2):257-64. https://doi.org/10.1016/S0008-6363(98)00251-X
30. Lombardi F. Chaos theory, heart rate variability, and arrhythmic mortality. Circulation. 2000;101(1):8-10. https://doi.org/10.1161/01.CIR.101.1.8
31. Kunz VC, Souza RB, Takahashi AC, Catai AM, Silva E. The relationship between cardiac autonomic function and clinical and angiographic characteristics in patients with coronary artery disease. Rev Bras Fisioter. 2011;15(6):503-10. https://doi.org/10.1590/S1413-35552011005000020
32. Bär KJ, Boettger MK, Koschke M, Schulz S, Chokka P, Yeragani VK, et al. Non-linear complexity measures of heart rate variability in acute schizophrenia. Clin Neurophysiol. 2007;118(9):2009-15. https://doi.org/10.1016/j.clinph.2007.06.012
33. Takahashi AC, Porta A, Melo RC, Quitério RJ, da Silva E, Borghi-Silva A, et al. Aging reduces complexity of heart rate variability assessed by conditional entropy and symbolic analysis. Intern Emerg Med. 2012;7(3):229-35. https://doi.org/10.1007/s11739-011-0512-z
34. Bernardo AF, Rossi RC, Souza NM, Pastre CM, Vanderlei LC. Association between physical activity and cardiovascular risk factors in individuals undergoing cardiac rehabilitation program Rev Bras Med Esporte. 2013;19(4):231-5. https://doi.org/10.1590/S1517-86922013000400001
35. Handa R, Poanta L, Rusu D, Albu A. The role of heart rate variability in assessing the evolution of patients with chronic obstructive pulmonary disease. Rom J Intern Med. 2012;50(1):83-8.
36. Reis MS, Arena R, Archiza B, de Toledo CF, Catai AM, Borghi-Silva A. Deep breathing heart rate variability is associated with inspiratory muscle weakness in chronic heart failure. Physiother Res Int. 2014;19(1):16-24. https://doi.org/10.1002/pri.1552
37. Volterrani M, Scalvini S, Mazzuero G, Lanfranchi P, Colombo R, Clark AL, et al. Decreased heart rate variability in patients with chronic obstructive pulmonary disease. Chest. 1994;106(5):1432-7. https://doi.org/10.1378/chest.106.5.1432
38. Bernardo AF, Vanderlei LC, Garner DM. HRV Analysis : A Clinical and Diagnostic Tool in Chronic Obstructive Pulmonary Disease. Int Sch Res Notices. 2014;2014:673232. https://doi.org/10.1155/2014/673232
39. Mazzuco A, Medeiros WM, Sperling MP, de Souza AS, Alencar MC, Arbex FF, et al. Relationship between linear and nonlinear dynamics of heart rate and impairment of lung function in COPD patients. Int J Chron Obstruct Pulmon Dis. 2015;10:1651-61. https://doi.org/10.2147/COPD.S81736
40. PAGANI M, LUCINI D, PIZZINELLI P, SERGI M, BOSISIO E, MELA GS, ET AL. EFFECTS OF AGING AND OF CHRONIC OBSTRUCTIVE PULMONARY DISEASE ON RR INTERVAL VARIABILITY. J AUTON NERV SYST. 1996;59(3):125-32. HTTPS://DOI.ORG/10.1016/0165-1838(96)00015-X