ABSTRACT
Objective: To determine the relationships that smoking history has with inflammatory markers, metabolic markers, body composition, muscle strength, and cardiopulmonary capacity in current smokers. Methods: This was a cross-sectional study involving 65 smokers (age range: 18-60 years). On three non-consecutive days, each participant was evaluated in terms of smoking history, pre-existing comorbidities, lung function (by spirometry), peripheral muscle strength (by dynamometry), body composition (by bioelectrical impedance analysis), levels of metabolic/inflammatory markers, and maximum cardiopulmonary capacity (by treadmill exercise test). We evaluated the relationships that smoking history has with inflammatory markers, metabolic markers, body composition, muscle strength, and cardiopulmonary capacity, using logarithmic transformation of the data and calculating Pearson's correlation coefficient and for partial correlations adjusted for age, gender, body mass index (BMI), and comorbidities. To identify the influence of smoking history on pre-existing comorbidities, we used a logistic regression model adjusted for age, BMI, and duration of smoking. Results: Smoking history correlated significantly, albeit weakly, with triglyceride level (r = 0.317; p = 0.005), monocyte count (r = 0.308; p = 0.013), and waist circumference (r = 0.299; p = 0.017). However, those correlations did not retain their significance in the adjusted analysis. In the logistic regression model, smoking more than 20 cigarettes/day correlated significantly with the presence of metabolic diseases (OR = 0.31; 95% CI: 1.009-1.701; p = 0.043). Conclusions: In this sample of smokers, smoking history correlated positively with the triglyceride level, the monocyte count, and waist circumference. The prevalence of metabolic disease was highest in those who smoked more than 20 cigarettes/day.
Keywords:
Tobacco; Smoking; Triglycerides; Monocytes; Waist circumference; Body composition.
RESUMO
Objetivo: Verificar a relação da carga tabágica com marcadores inflamatórios, marcadores metabólicos, composição corporal, força muscular e capacidade cardiorrespiratória em tabagistas. Métodos: Estudo transversal com 65 tabagistas de ambos os sexos (idade: 18-60 anos). Todos os participantes foram avaliados em três dias não consecutivos quanto ao histórico de tabagismo, comorbidades pré-existentes, função pulmonar (espirometria), força muscular periférica (dinamometria), composição corporal (bioimpedância), dosagem de marcadores metabólicos e inflamatórios e teste cardiopulmonar em esteira para avaliar a capacidade cardiorrespiratória máxima. Avaliou-se a relação da carga tabágica com marcadores inflamatórios, marcadores metabólicos, composição corporal, força muscular e capacidade cardiorrespiratória com transformação logarítmica através da correlação de Pearson e correlações parciais ajustadas para idade, sexo, índice de massa corpórea (IMC) e comorbidades. A regressão logística com modelo ajustado para idade, IMC e tempo de tabagismo foi utilizada para identificar a influência do histórico de tabagismo sobre as comorbidades pré-existentes. Resultados: Observaram-se correlações positivas fracas somente para dados não ajustados da carga tabágica com nível de triacilglicerol (r = 0,317; p = 0,005), contagem de monócitos (r = 0,308; p = 0,013) e circunferência abdominal (r = 0,299; p = 0,017). No modelo de regressão logística, fumar mais de 20 cigarros/dia correlacionou-se significativamente com a presença de doenças metabólicas (OR = 0,31; IC95%: 1,009-1,701; p = 0,043). Conclusões: Nesta amostra de tabagistas, a carga tabágica se correlacionou positivamente com nível de triacilglicerol, contagem de monócitos e circunferência abdominal. A prevalência de doenças metabólicas foi maior em tabagistas que fumam mais de 20 cigarros/dia.
Palavras-chave:
Tabaco; Fumar; Triglicerídeos; Monócitos; Circunferência abdominal; Composição corporal.
INTRODUCTIONSmoking is considered a chronic disease.(1) Tobacco use causes more than 7 million deaths per year(2) and is responsible for the development of various conditions,(3) such as atherosclerotic diseases, which cause mild inflammation and can lead to dyslipidemias(4) and sarcopenia through a catabolic response of the skeletal muscles.(5)
Smoking affects the lipid profile, reducing the level of HDL, which is cardioprotective, as well as increasing total cholesterol, LDL, and triglyceride levels.(6) As a consequence, it causes endothelial dysfunction, oxidative stress, dyslipidemias, atherosclerosis, and cardiovascular diseases.(6)
Smoking can also change body composition, which, in turn, affects glucose homeostasis, diminishing pancreatic β-cell function and leading to insulin resistance. This process negatively influences the waist-to-hip ratio and the visceral fat profile of smokers, predictors of morbidity and mortality(7) that are hallmarks of metabolic syndrome, which results in arterial hypertension, insulin resistance, abnormal cholesterol levels, and fat deposition in the abdominal region.(8)
Smoking is an external agent that attacks the respiratory system, leading to an increase in local defense cells, such as monocytes, lymphocytes, eosinophils, leukocytes, and neutrophils.(9) Chronic smoking affects the functioning of the peripheral immune system and can lead to low-grade chronic inflammation.(9) In addition, it can predispose an individual to the development of COPD, generating an abnormal inflammatory response in the lungs, loss of skeletal muscle mass, and muscle dysfunction.(10) The proteolysis triggered by tobacco use results in muscle atrophy and a consequent decrease in cardiopulmonary capacity.(10,11)
The magnitude of the negative impact of smoking is related to what is known as the smoking history. Although smoking fewer cigarettes per day for a longer period of time has been shown to be more harmful than is smoking more cigarettes per day for a shorter period of time,(12) calculating the number of pack-years is still considered an appropriate way to investigate the relationship between smoking history and the risk profile in this population.
The objective of the present study was to evaluate the relationships that smoking history has with inflammatory markers, metabolic markers, body composition, and muscle strength, as well as cardiopulmonary capacity, in current smokers.
METHODSWe evaluated 65 smokers between 18 and 60 years of age (Figure 1). All of the procedures performed in this study were approved by the Research Ethics Committee of the School of Science and Technology of São Paulo State University, Presidente Prudente Campus (Reference no. 53299816.9.0000.5402). All participants gave written informed consent.
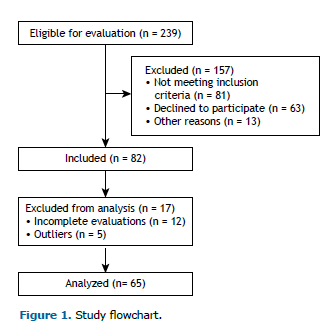
The study included smokers who were clinically stable and had a body mass index (BMI) < 40 kg/m2. Individuals who did not complete all evaluations were excluded from the study, as were those who had changed their medication in the last 30 days, those who had a pre-existing chronic respiratory diseases (FEV1/FVC ratio < 0.7), those who had cancer, those who had an uncontrolled cardiac or metabolic disease, and those who used nicotine replacement therapy or antidepressants as smoking cessation aids.
Study designThis was a cross-sectional study in which participants were evaluated on three non-consecutive days. All tests were done in the morning in a temperature- and humidity-controlled environment (22.0°C ± 2.2°C and 56.6% ± 6.9%, respectively). Participants were instructed not to consume tobacco, alcohol, caffeine, analgesics, or barbiturates 12 h before the evaluations. All evaluations were overseen by specialized professionals.
On the first day of evaluations, participant histories were taken and personal data (gender, age, weight, and height) were collected, as were data related to smoking (duration of smoking, number of cigarettes/day, smoking history, and level of nicotine dependence) and pre-existing comorbidities (cardiovascular or metabolic diseases such as systemic arterial hypertension, type 2 diabetes mellitus, and dyslipidemia). On the same day, lung function was evaluated by spirometry and peripheral muscle strength was evaluated by dynamometry. On the second day, body composition was evaluated by bioelectrical impedance analysis and peripheral venous blood was drawn for biochemical analysis. On the third day, each participant underwent a treadmill exercise test to determine maximum cardiopulmonary capacity and maximum oxygen consumption (VO2max).
ProceduresEach participant answered questions about the number of cigarettes smoked per day and duration of smoking. Their level of nicotine dependence was assessed with the Fagerström test,(13) which classifies nicotine dependence into five levels(14): very low (0 to 2 points); low (3 to 4 points); moderate (5 points); high (6 to 7 points); and very high (8 to 10 points). For each participant, the smoking history (pack-years) was calculated with the following formula: the number of cigarettes smoked per day divided by 20 and multiplied by the number of years of smoking.
The level of exhaled carbon monoxide was measured with a portable carbon monoxide monitor (Micro CO; Micro Medical Ltd., Rochester, England). Participants were instructed to take a deep breath and hold it for 15-20 s and then exhale slowly and constantly.(15) The exhaled carbon monoxide level was measured before the other evaluations to make sure that they had gone 12 h without smoking. The cut-off point to be achieved in order to go on to the other tests was 10 ppm.(16)
Lung function was evaluated with a portable MIR-Spirobank spirometer, version 3.6 (Medical International Research, Rome, Italy). The results were interpreted in accordance with the standards of the American Thoracic Society/European Respiratory Society,(17) and we employed reference values for the Brazilian population.(18) The lung function pattern was established in relation to the lower limit of normal (LLN) for an obstructive pattern (FEV1/FVC ratio < LLN and FVC ≥ LLN), a restrictive pattern (FEV1/FVC ratio ≥ LLN and FVC < LLN), and a mixed pattern (FEV1/FVC ratio < LLN and FVC < LLN).(19)
An electronic dynamometer (Power Din Standard; CEFISE, São Paulo, Brazil) was used in order to estimate peripheral muscle strength for the muscle groups responsible for the following movements(20): shoulder flexion, elbow flexion, shoulder abduction, knee extension, and knee flexion. Each test was performed three times, with specific dynamometer cables connected to the load cell and to the computer, and a one-minute interval between each attempt. Peak force and mean force were measured (in kgf). The highest values obtained for each variable and segment were recorded for analysis.
The InBody 720 device (InBody, Cerritos, CA, USA) was used for assessing body composition, including weight, BMI, body fat percentage, muscle mass, fat mass, waist circumference, and waist-to-hip ratio. The device has eight electrodes: four for each hand-in contact with the palm (E1 and E3) and thumb (E2 and E4); and four for each foot-in contact with the sole (E5 and E7) and heel (E6 and E8).(21,22) Participants were instructed to fast for 12 h and not to engage in moderate or vigorous exercise for 24 h before the evaluation.
After the 12-h fast, 14-mL samples of peripheral venous blood were collected by qualified professionals using disposable and sterilized materials. The blood specimens were collected into three vacuum tubes, centrifuged for 15 min at 3,500 rpm to separate the product to be analyzed and have the levels of triglyceride, total cholesterol, HDL, LDL, and glucose determined. The biological material was stored at −70°C, in accordance with the manufacturer's instructions. Glucose and lipid profile analyses were run on a spectrophotometer (SpectraMax Plus 384; Molecular Devices LLC, San Jose, CA, USA). Complete blood count and platelet count were performed by a specialized laboratory in an automated hematology analyzer (STKS; Coulter Electronics of Canada, Burlington, Canada).
For the evaluation of the maximal functional capacity, participants were submitted to a treadmill exercise test at an initial speed of 5.0 km/h, a constant incline of 1%, and speed increases of 0.5 km/h every 2 min. The test was continued until voluntary fatigue (VO2max).(23) The HR and SpO2 were monitored continuously; the subjective perception of exertion was rated according to the Borg dyspnea scale. In addition, the ventilatory variables were determined breath by breath with a pulmonary function testing system (Quark PFT; Cosmed, Rome, Italy), which was previously calibrated for each test in accordance with the manufacturer's specifications.
The VO2max was presumed to be the highest mean oxygen consumption in the last 30 s of exercise, when at least two of the following three criteria were met: HR > 90% of the age-predicted maximum (220 − age); respiratory exchange ratio > 1.10; and variation in VO2 between the penultimate and final stages of the exercise < 2.1 mL/kg per min. If a participant became fatigued before the end of the stage, the VO2max was calculated with the following equation:
VO2peak = Scom + (t/180) × I
where Scom stands for the last stage completed by the participant; t stands for the time spent in the last incomplete load (the duration of each stage being 180 s); and I stands for the speed increment (0.5 km/h).
Statistical analysisWe tested the data for normality with the Shapiro-Wilk test and found that they had a non-normal distribution. The data were therefore expressed as medians and interquartile ranges (IQRs). We evaluated the relationships that smoking history had with inflammatory markers, metabolic markers, body composition, muscle strength, and cardiopulmonary capacity, using logarithmic transformation to decrease the variability of the nonparametric variables, except for qualitative data, such as gender and comorbidities. For those data, we used Pearson's correlation coefficient and partial correlations adjusted for age, gender, BMI, and comorbidities. The responses were classified (by correlation coefficient) as weak (< 0.4), moderate (≥ 0.4 and < 0.5), or strong (≥ 0.5).(24) We then used a linear regression model adjusted for the same confounders.
The logistic regression model adjusted for age, BMI, and duration of smoking was used in order to identify the influence of smoking history on pre-existing comorbidities. The participants were divided into two groups: those who smoked ≤ 20 cigarettes/day (n = 12) and those who smoked > 20 cigarettes/day (n = 53). Comorbidities were classified as present (1) or absent (0). The increase in relative risk was calculated with the following formula: [Exp(B) - 1] × 100. All analyses were carried out with the Statistical Package for the Social Sciences, version 15.0 (SPSS Inc., Chicago, IL, USA). A significance level of 5% was used for all tests.
RESULTSTable 1 shows the general characteristics of our sample (N = 65), including smoking history, as well as demographic, anthropometric, and lung function data. Based on the LLN, 57 participants (88%) had a normal spirometry pattern, whereas 2 (3%) had an obstructive pattern, 4 (6%) had a restrictive pattern, and 2 (3%) had a mixed pattern.
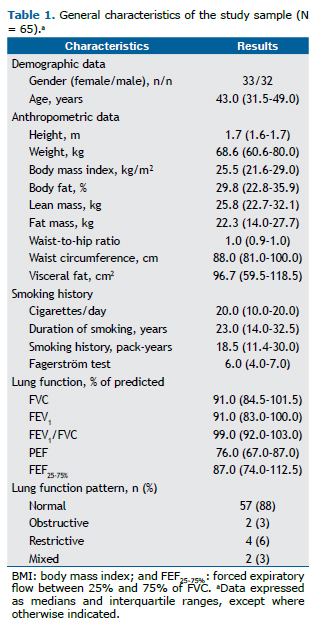
Table 2 shows the data on inflammatory markers, metabolic markers, muscle strength, cardiopulmonary capacity, and the presence of comorbidities in our study sample. All of the participants with cardiovascular disease had systemic arterial hypertension. Of the 6 participants with metabolic disease, 5 had dyslipidemia and 1 had type 2 diabetes mellitus.
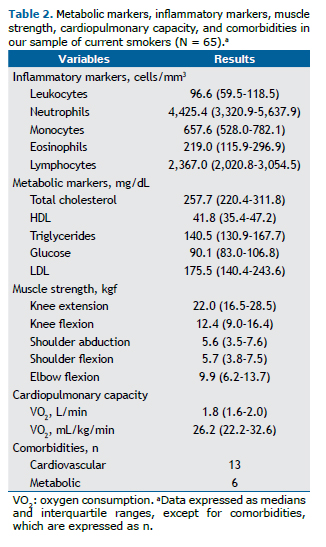
Figure 2 shows the analyses of the Pearson's correlation coefficients, in which we found that smoking history showed weak positive correlations with the triglyceride level (r = 0.317; p = 0.005), monocyte count (r = 0.308; p = 0.013), and abdominal circumference (r = 0.299; p = 0.017). No significant correlations were found between smoking history and the covariates in the partial correlations adjusted for age, gender, BMI, and comorbidities.
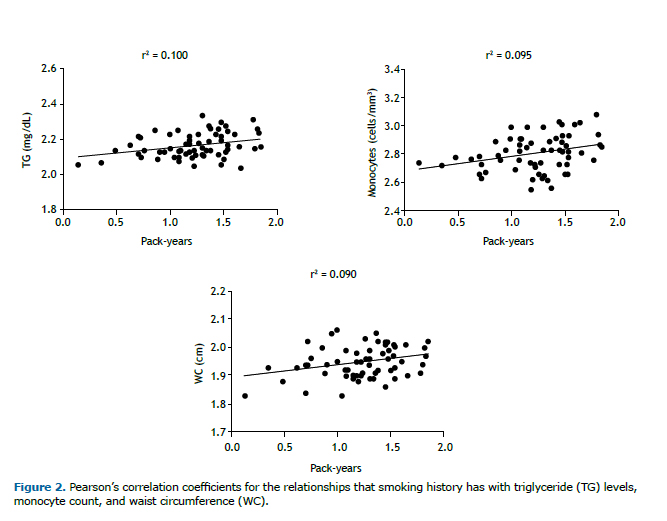
Finally, the logistic regression revealed that participants who smoked more than 20 cigarettes/day had an OR of 3.1 for metabolic diseases when compared with participants who smoked fewer than 20 cigarettes/day (p = 0.043; 95% CI: 1.009-1.701).
DISCUSSIONAlthough we found smoking history to correlate with monocyte count, triglyceride level, and waist circumference, the correlations were weak and did not withstand adjustment. Another important finding of our study was that participants who smoked more than 20 cigarettes/day were at a 31% higher risk of developing metabolic disease than were those who smoked fewer than 20 cigarettes/day.
Reynolds et al.(25) found that exposure to smoking causes changes in monocyte gene expression. When monocytes enter the subendothelial spaces of blood vessels, they can predispose the organism to the development of atherosclerosis. This happens because monocytes respond to chemotactic factors and start migrating and adhering to a layer of endothelial cells, potentially differentiating into macrophages thereafter. Those macrophages absorb low-density lipoproteins and turn into foam cells that gradually accumulate in the vessels, contributing to the development of atherosclerotic lesions.(26,27) Merianos et al.(28) found that adolescent smokers had increased levels of triglycerides. Consequently, such individuals are at an increased risk of atheromatous plaque formation.(27)
A study involving a sample of smokers and nonsmokers in Switzerland (all of whom were White and 35-75 years of age) identified a trend towards a dose-response effect between the number of cigarettes smoked and waist circumference.(29) In the present study, we also observed a weak positive correlation between smoking history and waist circumference. Waist circumference can also be associated with an increase in insulin resistance and have an indirect relationship with obesity, because an increased waist circumference is a marker for the development of diabetes, hypertension, metabolic syndrome, atherosclerosis, and cardiovascular diseases.(30,31)
According to a systematic review with meta-analysis,(32) a one-cigarette-per-day increase in consumption increases the waist circumference by 0.14% even when the BMI remains constant, that is, greater smoking intensity is associated with a propensity for central fat distribution. That may also explain the positive correlation that we found between waist circumference and smoking history, given that the magnitude of changes in the abdominal region is directly proportional to the number of cigarettes smoked.
In the present study, smoking history was not found to correlate with monocyte count, triglyceride level, or waist circumference after adjustments for gender, age, BMI, and comorbidities. Therefore, these factors may be interfering in this relationship in addition to the smoking history. Several factors can trigger the development of diseases; in this case, smoking is a modifiable risk factor, as are BMI and comorbidities, whereas gender and age are not.(33)
The fact that smoking history was not found to correlate with and lipid profile-related variables (total cholesterol, HDL, and LDL) corroborates the findings of a study conducted by Rom et al.,(34) who also found no such correlations. However, in a review of the literature, Chelland Campbell et al.(6) found that smokers have higher levels of lipid-profile related variables than nonsmokers. Contrary to the findings of our study, Marano et al.(35) found that the levels of inflammatory markers were higher in smokers than in nonsmokers, a finding that could be explained by their small sample size.
Our finding that smoking history did not correlate with muscle strength or cardiopulmonary capacity corroborates those of Wüst et al.(36) who found that, in the absence of COPD, smokers did not present muscle weakness or changes in the contractile properties of the quadriceps muscle, although they did show more fatigue. It is likely that this correlation was not seen in our study because our sample was composed of current smokers without COPD. However, we can presume that the individuals in our sample are at a higher risk of developing COPD and other diseases, with the consequent loss of muscle mass related to the systemic inflammation caused by COPD and to smoking itself, which activates protein degradation pathways.(11,37)
Our study has some limitations, including the small sample size and the fact that we did not measure cytokine levels, as well as the lack of a control group, which could have helped us understand the results in a more consistent way. Therefore, we suggest that further prospective studies be carried out to investigate the relationship between smoking history and the development of metabolic diseases, drawing comparisons between smokers and healthy nonsmokers without comorbidities, as well as between normal-weight and overweight individuals.
Smokers are at an increased risk of developing various diseases, especially metabolic disorders, which, once developed, can lead to the appearance of other diseases. In clinical practice, professionals should be alert to the accumulation of fat in the abdominal region and the number of cigarettes smoked per day, promoting smoking cessation or, when that is not possible, raising awareness about the importance of reducing the number of cigarettes smoked per day, engaging in physical activity, and having a more controlled, balanced diet.
In summary, we found a positive correlation between smoking history and triglyceride levels, monocyte count, and waist circumference. The prevalence of metabolic diseases was highest in those who smoked more than 20 cigarettes per day.
AUTHOR CONTRIBUTIONSAll authors made significant individual contributions to the development of this study. TSG, IBT, CPS, MP, and DR contributed to the study conception and design; TSG and CPS collected the data; TSG, IBT, and BSAS analyzed and interpreted the data; IBT conducted the statistical analysis; TSG, IBT, CPS, MP, and DR secured the research funding; and all authors participated in the writing of the manuscript and critical review of the intellectual content.
REFERENCES1. Mantoani LC, Furlanetto KC, Kovelis D, Proenca M, Zabatiero J, Bisca G, et al. Long-term effects of a program to increase physical activity in smokers. Chest. 2014;146(6):1627-1632. https://doi.org/10.1378/chest.14-0459
2. World Health Organization [homepage on the Internet]. Geneva: WHO; [cited 2017 Sep 27]. World No Tobacco Day. World Available from: http://www.who.int/campaigns/no-tobacco-day/2017/event/en/
3. Banks E, Joshy G, Weber MF, Liu B, Grenfell R, Egger S, et al. Tobacco smoking and all-cause mortality in a large Australian cohort study: findings from a mature epidemic with current low smoking prevalence. BMC Med. 2015;13:38. https://doi.org/10.1186/s12916-015-0281-z
4. Nagao Y, Hirayama S, Kon M, Sasamoto K, Sugihara M, Hirayama A, et al. Current smokers with hyperlipidemia lack elevated preβ1-high-density lipoprotein concentrations. J Clin Lipidol. 2017;11(1):242-249. https://doi.org/10.1016/j.jacl.2016.12.012
5. Rom O, Reznick AZ. The role of E3 ubiquitin-ligases MuRF-1 and MAFbx in loss of skeletal muscle mass. Free Radic Biol Med. 2016;98:218-230. https://doi.org/10.1016/j.freeradbiomed.2015.12.031
6. Chelland Campbell S, Moffatt RJ, Stamford BA. Smoking and smoking cessation -- the relationship between cardiovascular disease and lipoprotein metabolism: a review. Atherosclerosis. 2008;201(2):225-235. https://doi.org/10.1016/j.atherosclerosis.2008.04.046
7. Rinaldi M, Maes K, De Vleeschauwer S, Thomas D, Verbeken EK, Decramer M, et al. Long-term nose-only cigarette smoke exposure induces emphysema and mild skeletal muscle dysfunction in mice. Dis Model Mech. 2012;5(3):333-341. https://doi.org/10.1242/dmm.008508
8. Balhara YPS, Kalra S, Bajaj S, Kuppili PP, Himanshu D, Atam V, et al. Uttar Pradesh Association of Physicians of India Position Statement: Tobacco Use and Metabolic Syndrome. J Assoc Physicians India. 2017;65(12):66-72.
9. Yasue H, Hirai N, Mizuno Y, Harada E, Itoh T, Yoshimura M, et al. Low-grade inflammation, thrombogenicity, and atherogenic lipid profile in cigarette smokers. Circ J. 2006;70(1):8-13. https://doi.org/10.1253/circj.70.8
10. Krüger K, Seimetz M, Ringseis R, Wilhelm J, Pichl A, Couturier A, et al. Exercise training reverses inflammation and muscle wasting after tobacco smoke exposure. Am J Physiol Regul Integr Comp Physiol. 2018;314(3):R366-R376. https://doi.org/10.1152/ajpregu.00316.2017
11. Plant PJ, Brooks D, Faughnan M, Bayley T, Bain J, Singer L, et al. Cellular markers of muscle atrophy in chronic obstructive pulmonary disease. Am J Respir Cell Mol Biol. 2010;42(4):461-471. https://doi.org/10.1165/rcmb.2008-0382OC
12. Lubin JH, Couper D, Lutsey PL, Yatsuya H. Synergistic and Non-synergistic Associations for Cigarette Smoking and Non-tobacco Risk Factors for Cardiovascular Disease Incidence in the Atherosclerosis Risk In Communities (ARIC) Study. Nicotine Tob Res. 2017;19(7):826-835. https://doi.org/10.1093/ntr/ntw235
13. Heatherton TF, Kozlowski LT, Frecker RC, Fagerström KO. The Fagerström Test for Nicotine Dependence: a revision of the Fagerström Tolerance Questionnaire. Br J Addict. 1991;86(9):1119-1127. https://doi.org/10.1111/j.1360-0443.1991.tb01879.x
14. Meneses-Gaya IC, Zuardi AW, Loureiro SR, Crippa JA. Psychometric properties of the Fagerström Test for Nicotine Dependence. J Bras Pneumol. 2009;35(1):73-82. https://doi.org/10.1590/S1806-37132009000100011
15. Santos UP, Gannam S, Abe JM, Esteves PB, Freitas Filho M, Wakassa TB, et al. Emprego da determinação de monóxido de carbono no ar exalado para a detecção do consumo de tabaco. J Pneumol. 2001;27(5):231-236. https://doi.org/10.1590/S0102-35862001000500001
16. SRNT Subcommittee on Biochemical Verification. Biochemical verification of tobacco use and cessation. Nicotine Tob Res. 2002;4(2):149-159. https://doi.org/10.1080/14622200210123581
17. Miller MR, Hankinson J, Brusasco V, Burgos F, Casaburi R, Coates A, et al. Standardisation of spirometry. Eur Respir J. 2005;26(2):319-338. https://doi.org/10.1183/09031936.05.00034805
18. Duarte AA, Pereira CA, Rodrigues SC. Validation of new Brazilian predicted values for forced spirometry in Caucasians and comparison with predicted values obtained using other reference equations. J Bras Pneumol. 2007;33(5):527-535. https://doi.org/10.1590/S1806-37132007000500007
19. Pellegrino R, Viegi G, Brusasco V, Crapo RO, Burgos F, Casaburi R, et al. Interpretative strategies for lung function tests. Eur Respir J. 2005;26(5):948-968. https://doi.org/10.1183/09031936.05.00035205
20. Melchiorri G, Rainoldi A. Muscle fatigue induced by two different resistances: Elastic tubing versus weight machines. J Electromyogr Kinesiol. 2011;21(6):954-959. https://doi.org/10.1016/j.jelekin.2011.07.015
21. Janssen I, Heymsfield SB, Ross R. Low relative skeletal muscle mass (sarcopenia) in older persons is associated with functional impairment and physical disability. J Am Geriatr Soc. 2002;50(5):889-896. https://doi.org/10.1046/j.1532-5415.2002.50216.x
22. Cunningham JJ. Body composition as a determinant of energy expenditure: a synthetic review and a proposed general prediction equation. Am J Clin Nutr. 1991;54(6):963-969. https://doi.org/10.1093/ajcn/54.6.963
23. Midgley AW, Bentley DJ, Luttikholt H, McNaughton LR, Millet GP. Challenging a dogma of exercise physiology: does an incremental exercise test for valid VO 2 max determination really need to last between 8 and 12 minutes?. Sports Med. 2008;38(6):441-447. https://doi.org/10.2165/00007256-200838060-00001
24. Hulley SB, Cumming SR, Browner WS, Grady D, Hearst N, Newman TB. Delineando a pesquisa clínica: uma abordagem epidemiológica. 2nd ed. Porto Alegre: Artmed; 2003.
25. Reynolds LM, Lohman K, Pittman GS, Barr RG, Chi GC, Kaufman J, et al. Tobacco exposure-related alterations in DNA methylation and gene expression in human monocytes: the Multi-Ethnic Study of Atherosclerosis (MESA). Epigenetics. 2017;12(12):1092-1100. https://doi.org/10.1080/15592294.2017.1403692
26. Libby P, Ridker PM, Hansson GK. Progress and challenges in translating the biology of atherosclerosis. Nature. 2011;473(7347):317-325. https://doi.org/10.1038/nature10146
27. van der Toorn M, Frentzel S, Goedertier D, Peitsch M, Hoeng J, De Leon H. A prototypic modified risk tobacco product exhibits reduced effects on chemotaxis and transendothelial migration of monocytes compared with a reference cigarette. Food Chem Toxicol. 2015;80:277-286. https://doi.org/10.1016/j.fct.2015.03.026
28. Merianos AL, Jandarov RA, Khoury JC, Mahabee-Gittens EM. Tobacco Smoke Exposure Association With Lipid Profiles and Adiposity Among U.S. Adolescents. J Adolesc Health. 2018;62(4):463-470.
29. Clair C, Chiolero A, Faeh D, Cornuz J, Marques-Vidal P, Paccaud F, et al. Dose-dependent positive association between cigarette smoking, abdominal obesity and body fat: cross-sectional data from a population-based survey. BMC Public Health. 2011;11:23. https://doi.org/10.1186/1471-2458-11-23
30. García Álvarez A, Serra-Majem L, Castell C, Ribas-Barba L, Méndez MA. Trends in the association between smoking history and general/central obesity in Catalonia, Spain (1992-2003). Nutr Hosp. 2017;34(1):102-110. https://doi.org/10.20960/nh.984
31. Elffers TW, de Mutsert R, Lamb HJ, de Roos A, Willems van Dijk K, Rosendaal FR, et al. Body fat distribution, in particular visceral fat, is associated with cardiometabolic risk factors in obese women. PLoS One. 2017;12(9):e0185403. https://doi.org/10.1371/journal.pone.0185403
32. Morris RW, Taylor AE, Fluharty ME, Bjorngaard JH, Asvold BO, Elvestad Gabrielsen M, et al. Heavier smoking may lead to a relative increase in waist circumference: evidence for a causal relationship from a Mendelian randomisation meta-analysis. The CARTA consortium [published correction appears in BMJ Open. 2015;5(9):e008808]. BMJ Open. 2015;5(8):e008808. https://doi.org/10.1136/bmjopen-2015-008808corr1
33. Altaleb FF, Alshammari OM, Alanazi HM, Aljaber DA, Alanazi AB, El-Fetoh NMA, et al. Pattern and factors associated with cardiovascular diseases among patients attending the cardiac center in Arar City, Northern Saudi Arabia. Electron Physician. 2017;9(10):5459-5464. https://doi.org/10.19082/5459
34. Rom O, Karkabi K, Reznick AZ, Keidar Z, Aizenbud D. Relationship between history of smoking, metabolic and inflammatory markers, parameters of body composition and muscle strength. Adv Exp Med Biol. 2015;849:49-56. https://doi.org/10.1007/5584_2014_92
35. Marano KM, Kathman SJ, Jones BA, Nordskog BK, Brown BG, Borgerding MF. Study of cardiovascular disease biomarkers among tobacco consumers. Part 3: evaluation and comparison with the US National Health and Nutrition Examination Survey. Inhal Toxicol. 2015;27(3):167-173. https://doi.org/10.3109/08958378.2015.1009196
36. Wüst RC, Morse CI, de Haan A, Rittweger J, Jones DA, Degens H. Skeletal muscle properties and fatigue resistance in relation to smoking history. Eur J Appl Physiol. 2008;104(1):103-110. https://doi.org/10.1007/s00421-008-0792-9
37. Abdulai RM, Jensen TJ, Patel NR, Polkey MI, Jansson P, Celli BR, et al. Deterioration of Limb Muscle Function during Acute Exacerbation of Chronic Ob-structive Pulmonary Disease. Am J Respir Crit Care Med. 2018;197(4):433-449. https://doi.org/10.1164/rccm.201703-0615CI