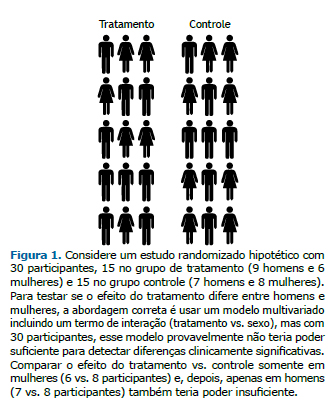
Um ensaio clínico randomizado foi conduzido para comparar o efeito da vitamina C vs. placebo na melhora da função pulmonar em recém-nascidos de fumantes grávidas; e para testar se esse efeito diferiu pelo genótipo materno.(1) A vitamina C melhorou a função pulmonar em recém-nascidos em comparação com o placebo (relação TPTEF:TE 0,383 vs. 0,345; p = 0,006); e este efeito foi mais forte em recém-nascidos com mães com um genótipo específico (p-interação < 0,001).(1)
CONTEXTOAo realizar ensaios clínicos, os investigadores examinam o efeito das intervenções sobre os desfechos na população estudada e muitas vezes em subgrupos de pacientes definidos pelas características basais (por ex., dados demográficos, fatores prognósticos). O objetivo é entender se a magnitude do efeito da intervenção difere nas categorias de um subgrupo; em nosso exemplo, os subgrupos de genótipos. Se o efeito for diferente nos subgrupos, chamamos isso de efeito modificador da intervenção no desfecho, devido à presença adicional da variável do subgrupo. Geralmente realizamos um teste de interação, usando modelos multivariados, para avaliar diferenças de subgrupo estatisticamente significantes. Se o valor de p for significativo, concluímos que o efeito da intervenção no desfecho difere nos subgrupos, em nosso exemplo, o genótipo materno.
Compreender os efeitos do tratamento em subgrupos de pacientes é importante porque ajuda a identificar grupos de pacientes que respondem melhor ou pior à intervenção. No entanto, as análises de subgrupos devem ser feitas com cautela para evitar erros comuns que resultam em achados falso-negativos ou positivos, especialmente quando não são pré-especificados no plano de análise antes de iniciar o estudo. Um erro comum é comparar o efeito do tratamento no desfecho separadamente em cada subgrupo. Por exemplo, comparar o efeito da vitamina C vs. placebo na função pulmonar em recém-nascidos entre mães com um genótipo e, em seguida, separadamente entre as mães com outro genótipo. Esta abordagem é incorreta porque leva a testes múltiplos, o que significa que, em vez de usar apenas um cálculo para testar as diferenças de efeito entre subgrupos (p para interação em grupos de genótipos em nosso exemplo), usamos dois ou mais cálculos diferentes para cada análise de subgrupos. Toda vez que adicionamos um cálculo, já não podemos usar o nível de significância padrão de p < 0,05. Neste caso, uma vez que existem dois cálculos, precisamos dividir o valor de p por 2 e usar p < 0,025 como o nível de significância.(2) Assim, nós iríamos superestimar as diferenças de subgrupos se mantivéssemos o nível de significância em 0,05. Outro desafio com a análise de subgrupos é que os resultados podem sugerir que existem diferenças entre subgrupos, mas o valor de p não é estatisticamente significativo porque o tamanho da amostra em cada subgrupo é muito pequeno (Figura 1).
DICAS DE ANÁLISE DE SUBGRUPOS1. Identifique alguns subgrupos que pareçam altamente relevantes para a sua pergunta do estudo a priori e justifique suas escolhas.
2. Não compare os efeitos do tratamento vs. controle em cada subgrupo. Existem testes estatísticos específicos para determinar se existe uma interação entre o efeito do tratamento e as variáveis que definem os subgrupos, que são melhor realizados com o auxílio de um estatístico.
3. Antes de fazer mudanças na prática clínica, os resultados em subgrupos devem ser replicados em outros estudos.
REFERÊNCIAS1. McEvoy CT, Schilling D, Clay N, Jackson K, Go MD, Spitale P, et al. Vitamin C supplementation for pregnant smoking women and pulmonary function in their newborn infants: a randomized clinical trial. JAMA. 2014;311(20):2074-82. https://doi.org/10.1001/jama.2014.5217
2. Wang R, Lagakos SW, Ware JH, Hunter DJ, Drazen JM. Statistics in medicine--reporting of subgroup analyses in clinical trials. N Engl J Med. 2007;357(21):2189-94. https://doi.org/10.1056/NEJMsr077003