ABSTRACT
Objective: To compare the performance of Charlson Comorbidity Index (CCI) with those of the mental Confusion, Urea, Respiratory rate, Blood pressure, and age = 65 years (CURB-65) score and the Pneumonia Severity Index (PSI) as predictors of all-cause in-hospital mortality in patients with community-acquired pneumonia (CAP). Methods: This was a cohort study involving hospitalized patients with CAP between April of 2014 and March of 2015. Clinical, laboratory, and radiological data were obtained in the ER, and the scores of CCI, CURB-65, and PSI were calculated. The performance of the models was compared using ROC curves and AUCs (95% CI). Results: Of the 459 patients evaluated, 304 met the eligibility criteria. The all-cause in-hospital mortality rate was 15.5%, and 89 (29.3%) of the patients were admitted to the ICU. The AUC for the CCI was significantly greater than those for CURB-65 and PSI (0.83 vs. 0.73 and 0.75, respectively). Conclusions: In this sample of hospitalized patients with CAP, CCI was a better predictor of all-cause in-hospital mortality than were the PSI and CURB-65.
Keywords:
Pneumonia, ROC curve; Predictive value of tests; Severity of illness index.
RESUMO
Objetivo: Comparar o desempenho do Índice de Comorbidade de Charlson (ICC) com o do mental Confusion, Urea, Respiratory rate, Blood pressure, and age = 65 years (CURB-65, Confusão mental, Ureia, frequência Respiratória, Pressão arterial e idade = 65 anos) e do Pneumonia Severity Index (PSI, Índice de Gravidade da Pneumonia) como preditores de mortalidade hospitalar por qualquer causa em pacientes com pneumonia adquirida na comunidade (PAC). Métodos: Estudo de coorte com pacientes hospitalizados com PAC entre abril de 2014 e março de 2015. Dados clínicos, laboratoriais e radiológicos foram obtidos no PS, e o ICC, CURB-65 e PSI foram calculados. O desempenho dos modelos foi comparado por meio de curvas ROC e ASC (IC95%). Resultados: Dos 459 pacientes avaliados, 304 preencheram os critérios de elegibilidade. A taxa de mortalidade hospitalar por qualquer causa foi de 15,5%, e 89 (29,3%) dos pacientes foram admitidos na UTI. A ASC do ICC foi significativamente maior do que a do CURB-65 e do PSI (0,83 vs. 0,73 e 0,75, respectivamente). Conclusões: Nesta amostra de pacientes hospitalizados com PAC, o ICC foi um preditor melhor de mortalidade hospitalar por qualquer causa do que o PSI e o CURB-65.
Palavras-chave:
Pneumonia; Curva ROC; Valor preditivo dos testes; Índice de gravidade de doença.
INTRODUÇÃO A pneumonia adquirida na comunidade (PAC) ainda é a principal causa de morte por doenças infecciosas no mundo,(1) com incidência anual de 5-11 casos por 1.000 habitantes e grande impacto nos sistemas de saúde.(2) Nos EUA, foram relatadas mais de 60.000 mortes por PAC em indivíduos com idade ≥ 15 anos em 2005, e a carga econômica anual ainda era elevada em 2010 (17 bilhões de dólares).(3)
A identificação precoce de pacientes com risco de morte é um princípio do manejo da PAC, sendo a definição da gravidade da doença o aspecto mais importante que orienta a decisão de internação hospitalar.(4,5) No entanto, é possível que a avaliação clínica não capture com precisão a gravidade da doença e o potencial de complicações ou morte.(5,6) Por isso, o uso de escores de gravidade(7-9) tem sido recomendado para avaliar pacientes com PAC e estabelecer a necessidade de terapia intensiva.
Entre os modelos de predição de risco de PAC mais conhecidos, o mental Confusion, Urea, Respiratory rate, Blood pressure, and age = 65 years (CURB-65, Confusão mental, Ureia, frequência Respiratória, Pressão arterial e idade = 65 anos)(10) e o Pneumonia Severity Index (PSI, Índice de Gravidade da Pneumonia),(11) cuja capacidade preditiva de mortalidade é de 0,79 e 0,82, respectivamente, foram validados para uso em diversos cenários clínicos. (12) No entanto, ambos os modelos dependem de critérios específicos da pneumonia e, portanto, não levam em consideração os riscos relacionados com comorbidades. Não obstante, estudos anteriores(13,14) mostram que informações sobre o número de comorbidades e o grau de comprometimento do estado de saúde são úteis para estabelecer o prognóstico. Nessa situação, um escore geral, como o Índice de Comorbidade de Charlson (ICC),(15) por exemplo, pode ser útil. O ICC, que foi desenvolvido para padronizar a avaliação de comorbidades(13) e mortalidade por qualquer causa em 1 e 10 anos,(15) é um preditor bem estabelecido de mortalidade hospitalar em pacientes não cirúrgicos(16) e naqueles com doenças específicas.(17) No entanto, ainda não foi investigado o uso do ICC para predizer a mortalidade hospitalar em pacientes com PAC, especialmente como uma alternativa aos escores de gravidade específicos para pneumonia. Assim, o objetivo do presente estudo foi comparar o desempenho do ICC com o do CURB-65 e do PSI como preditores de mortalidade hospitalar por qualquer causa em pacientes com PAC.
MÉTODOS População do estudo Este estudo foi realizado em um hospital geral comunitário com 130 leitos, na cidade de Montenegro (RS). O hospital oferece, pelo Sistema Único de Saúde, assistência à saúde de cerca de 160.000 habitantes em 19 cidades. A PAC foi o principal motivo de internação hospitalar, com taxa de mortalidade de aproximadamente 15,5%.(18) Quando o presente estudo foi realizado, o hospital estava começando a usar índices de gravidade para avaliar a necessidade de internação em pacientes que buscavam o PS. Os profissionais de saúde responsáveis pela coleta de dados padronizados para o cálculo dos índices foram treinados pelo grupo de pesquisa. Durante a fase de treinamento, 100% das avaliações foram realizadas em duplicata, o que gerou uma concordância geral interavaliadores de 96,3%.
Desenho do estudo No presente estudo de coorte, avaliamos pacientes com idade ≥ 14 anos que compareceram a nosso PS com queixas respiratórias entre abril de 2014 e março de 2015. Foram incluídos no estudo pacientes com diagnóstico clínico e radiográfico de PAC com necessidade de hospitalização. Foram excluídos pacientes com pneumonia nosocomial (caracterizada por admissão em unidades de atendimento de urgência por pelo menos 2 dias); pacientes provenientes de casas de repouso, abrigos ou outras instituições de assistência à saúde; pacientes que estivessem recebendo antibioticoterapia intravenosa ou quimioterapia; pacientes que tivessem recebido tratamento para lesões por pressão nos últimos 30 dias e pacientes que estivessem recebendo terapia renal substitutiva.
Os resultados da avaliação da gravidade da doença pelos modelos de predição de risco foram registrados nos prontuários médicos dos pacientes e tomados como dados basais da coorte. A evolução clínica dos pacientes foi avaliada durante a hospitalização. A alta hospitalar foi definida como medida de desfecho clínico.
A PAC foi diagnosticada com base em pelo menos um dos seguintes achados da TC de tórax: infiltrado novo ou progressivo, consolidação ou escavação; e pelo menos um dos seguintes sinais ou sintomas: febre > 38°C sem outra causa conhecida, leucopenia (< 4.000 leucócitos/mm3) ou leucocitose (≥ 12.000 leucócitos/mm3). Além disso, em pacientes com idade ≥ 70 anos, alteração do estado mental sem outra causa evidente e pelo menos dois dos seguintes foram considerados para o diagnóstico de PAC: tosse recente com escarro purulento, alteração da expectoração, aumento das secreções respiratórias, aumento da frequência de aspiração, surgimento ou agravamento de tosse, dispneia ou taquipneia, sibilância ou piora da troca gasosa [por exemplo, dessaturação de oxigênio (PaO2/FiO2 ≤ 240), maior necessidade de oxigênio ou necessidade de ventilação mecânica].
O estudo foi aprovado pelo conselho de revisão institucional (Protocolo n. 150168).
Variáveis do estudo Os dados clínicos, laboratoriais e radiológicos registrados no prontuário médico foram obtidos nas primeiras 24 h após a consulta no PS e incluíram idade, sexo, origem, FR, pressão arterial, temperatura, FC, presença de confusão mental, SpO2, comorbidades (incluídas no prontuário médico pelo médico assistente), histórico de internações hospitalares, achados da radiografia de tórax (relatados por um radiologista) e resultados de exames laboratoriais solicitados durante a visita ao PS. Os exames laboratoriais incluíram gasometria arterial, ureia, creatinina sérica, glicose, sódio e hemograma. Foram também coletadas informações sobre a duração da antibioticoterapia, tempo de internação hospitalar, tempo de internação na UTI e necessidade de ventilação mecânica. A principal medida de desfecho foi a mortalidade hospitalar por qualquer causa registrada no prontuário médico e confirmada pela análise do sumário de alta ou da declaração de óbito. Essas informações estavam disponíveis em relação a todos os pacientes com PAC tratados no hospital durante o estudo. O sumário de alta foi preparado pelo médico assistente após a alta em todos os casos.
Para a análise, os pacientes foram agrupados em duas categorias —risco baixo ou intermediário/alto — de acordo com o ponto de corte de cada modelo: o ICC(15) compreende 19 variáveis relacionadas a comorbidades, com pontuação de 1 a 6, sendo que pacientes com ICC = 0-2 são considerados pacientes com risco baixo de morte/internação; o CURB-65(10) baseia-se na avaliação de cinco características clínicas, com pontuação de 0 a 5, sendo que pacientes com pontuação = 0 ou 1 são considerados pacientes com risco baixo de morte/internação; o PSI(11) baseia-se em 20 variáveis clínicas para gerar uma pontuação com cinco classes representando aumento progressivo do risco de morte, sendo que pacientes com PSI = 1 ou 2 são considerados pacientes com risco baixo de morte.
Análise dos dados e cálculo do tamanho da amostra Para determinar a capacidade do ICC, CURB-65 e PSI de predizer o risco de morte, foram usadas curvas ROC e a estatística C (correspondente à ASC). A medida de calibração usada foi o teste de Hosmer-Lemeshow. Uma ASC = 0,5 indica ausência de poder discriminante; uma ASC = 0,7-0,8 indica utilidade clínica; valores acima de 0,8 indicam excelente capacidade preditiva. (19) As ASC foram comparadas por meio do método de DeLong para o ICC vs. CURB-65 e PSI. A comparação entre as proporções de pacientes considerados de risco baixo pelos três índices foi realizada por meio do teste de McNemar. Foi considerado estatisticamente significativo o valor de p bicaudal < 0,05. Uma análise bivariada das características clínicas vs. mortalidade foi realizada por meio do teste t de Student para médias e desvios-padrão ou do teste do qui-quadrado de Pearson para proporções.
Todas as análises foram realizadas com os programas IBM SPSS Statistics, versão 17 (SPSS Inc., Chicago, IL, EUA) e Epidat, versão 3.0 (Dirección Xeral de Saúde Pública de la Consellería de Sanidade, Xunta de Galicia, Santiago de Compostela, Espanha).
O tamanho da amostra foi calculado por meio de uma abordagem de simulação, considerando as diferenças entre os escores em termos de sensibilidade (variando de 75% a 95%), especificidade (de 50% a 70%), proporção sobrevida:morte = 6:1, poder estatístico de pelo menos 80% e IC95%. O tamanho da amostra resultante foi de 304 pacientes.
RESULTADOS Entre abril de 2014 e março de 2015, foram avaliados 459 pacientes com infecções respiratórias. Destes, 155 não preencheram os critérios diagnósticos de PAC, e 304 foram incluídos no estudo (Figura 1). A média de idade dos participantes foi de 67,1 ± 17,3 anos, 210 (69,0%) residiam em áreas urbanas, 171 (56,3%) eram do sexo masculino e 149 (49,0%) apresentavam asma ou DPOC como doença pulmonar preexistente. Durante o período de seguimento, 47 pacientes (15,5%) morreram, 89 (29,3%) foram admitidos na UTI e 98 (32,2%) necessitaram de ventilação mecânica (Tabela 1).
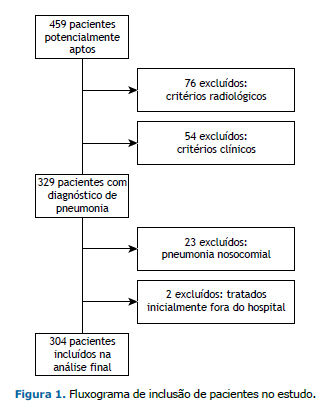
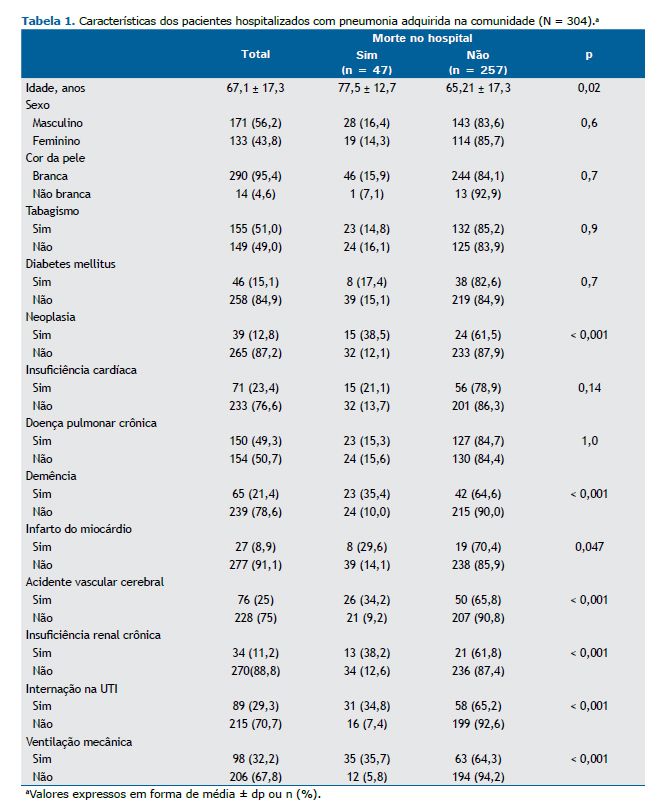
O exame clínico revelou que aproximadamente um terço dos participantes apresentava secreções nas vias aéreas, e o escarro foi coletado. Espécimes para cultura (escarro ou sangue) foram coletados de 203 pacientes (66,8%), e agentes infecciosos foram isolados em 52. O agente infeccioso mais comum foi Streptococcus pneumoniae, em 19 pacientes (36,5%). O tratamento baseou-se em amoxicilina + clavulanato (72,2%) e/ou azitromicina (65,6%). A média do tempo de internação hospitalar foi de 7,2 ± 7,4 dias (mediana: 5,0 dias).
A Tabela 2 mostra que a pontuação nos três modelos de predição de risco aumentou linearmente com o aumento da taxa de mortalidade. O número de pacientes considerados de risco baixo de acordo com o ICC, CURB-65 e PSI foi de 74 (24,3%), 89 (29,3%) e 80 (26,3%), respectivamente. A taxa de mortalidade de pacientes considerados de risco baixo pelo ICC, CURB-65 e PSI foi baixa (1,4%, 4,5% e 3,7%, respectivamente).
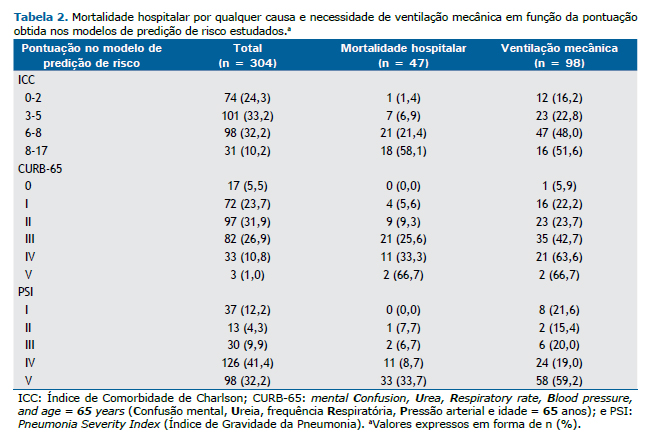
A Tabela 3 mostra que as ASC variaram de 0,73 a 0,84. O ICC apresentou a maior ASC, que foi significativamente diferente das ASC calculadas para o PSI (p = 0,04) e CURB-65 (p = 0,02). O ICC ≥ 3 e o PSI ≥ 3 foram capazes de detectar 93,6% dos pacientes com risco de morte no momento da admissão, ao passo que uma pontuação ≥ 2 no CURB-65 detectou 72,3% dos pacientes nessa categoria. Por outro lado, o PSI apresentou a menor especificidade, e o CURB-65 apresentou a maior especificidade para detectar pacientes com risco de morte no momento da admissão. Embora todos os modelos tenham apresentado valores preditivos positivos baixos, os valores preditivos negativos foram altos: a probabilidade de morte foi de 7,0% com pontuação < 2 no CURB-65, de 3,8% com PSI < 3 e de 2,2% com ICC < 3.
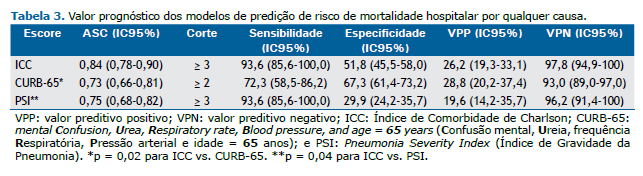
A Figura 2 mostra que o ICC foi um excelente preditor de mortalidade hospitalar por qualquer causa, com ASC maior (0,83) que a do CURB-65 (0,73; p = 0,02) e do PSI (0,75; p = 0,04). Não houve diferença estatística entre as ASC do CURB-65 e do PSI (p = 0,7). Após a calibração de Hosmer-Lemeshow, os valores de p para o ICC, PSI e CURB-65 foram 0,9988, 0,9769 e 0,9906, respectivamente.
Uma análise de sensibilidade comparando pacientes com e sem doença pulmonar prévia não revelou diferenças entre os modelos para predizer mortalidade hospitalar. O ICC de pacientes sem doença pulmonar prévia (0,86; IC95%: 0,78-0,93) foi semelhante ao daqueles com doença pulmonar prévia (0,82; IC95%: 0,73-0,91).
Em nosso estudo, decidimos não excluir pacientes com ordem de não reanimar (n = 29), e 24 deles morreram. Quando excluímos esses pacientes, não houve alterações importantes na ASC (ICC = 0,83; CURB-65 = 0,75; PSI = 0,74).
DISCUSSÃO O presente estudo com a estatística C mostrou que o ICC foi melhor do que o CURB-65 e o PSI para prever a mortalidade hospitalar por qualquer causa em pacientes hospitalizados por PAC. Pelo que sabemos, este foi o primeiro estudo no qual o ICC foi avaliado como preditor de mortalidade hospitalar por qualquer causa em pacientes com PAC que espontaneamente buscaram atendimento de emergência em um hospital comunitário ao longo de 1 ano.
Em um estudo anterior no qual o ICC foi comparado com o CURB-65 e o PSI, foram incluídos apenas idosos hospitalizados com pneumonia. O estudo não detectou diferença estatística entre os escores de predição de mortalidade ao longo de 1 ano.(20) As ASC observadas no presente estudo são semelhantes às descritas anteriormente para o CURB-65 (0,73 a 0,76) e o PSI (0,70 a 0,80).(11,21-24) É importante notar que os escores não medem o mesmo construto. O ICC é um escore de comorbidade, com diversas variáveis. Diferentemente do ICC, o CURB-65 e o CRB-65 (sem medição da ureia) são vistos como marcadores de gravidade da doença no momento da admissão semelhantes ao PSI. Nossos achados apoiam a noção de que, embora seja um escore geral, o ICC apresenta excelente desempenho preditivo em pacientes com PAC.
Pode-se relacionar o número de variáveis de um escore com seu desempenho geral; no entanto, apesar de incluir um número semelhante de variáveis, o ICC e o PSI diferem quanto às comorbidades, que são consideradas pelo ICC, ao passo que o PSI considera apenas as características específicas da pneumonia. Confirmamos a sensibilidade elevada do ICC e observamos uma proporção baixa de pacientes com PAC e ICC indicativo de risco baixo que morreram (1,4%). Esses achados sugerem que o ICC tem mais potencial para uso clínico do que o PSI ou o CURB-65.
O uso de modelos de predição de risco é respaldado por diretrizes de manejo da PAC.(7-9) No entanto, a gravidade da PAC é geralmente determinada por meio de avaliação clínica,(21) que é frequentemente realizada sem o apoio de uma ferramenta estruturada objetiva. (22) Nesse sentido, o ICC tem a vantagem de fazer parte da avaliação usual de gravidade em serviços de emergência e, consequentemente, não precisa ser introduzido na rotina de assistência ao paciente para avaliar indivíduos com pneumonia. Além disso, como não exige exames laboratoriais, o ICC é adequado para uso em contextos de emergência. Finalmente, o ICC já foi validado em diversos contextos clínicos, e os resultados obtidos até o momento mostram de forma consistente que o ICC é um bom preditor de mortalidade.(16,23,24) No presente estudo, a análise de sensibilidade mostrou que o desempenho prognóstico do ICC foi semelhante em pacientes com alta prevalência de doença pulmonar prévia.
Os resultados do presente estudo devem ser interpretados à luz de algumas limitações potenciais. Todos os participantes foram incluídos no estudo em um único centro em uma cidade de médio porte, o que poderia limitar a generalização dos achados em certa medida. Por outro lado, é provável que todos os pacientes aptos para inclusão tenham sido incluídos, já que o hospital comunitário é a única instituição onde pacientes com PAC podem ser internados naquela área geográfica. Outro aspecto positivo é que, durante o estudo, o ICC vinha sendo avaliado como estratégia institucional para a tomada de decisão a respeito da internação hospitalar. Isso se traduziu em engajamento institucional, padronização da avaliação clínica e elaboração de fichas clínicas para a coleta de dados, para uso no PS. Com isso, não houve perdas de seguimento e as informações coletadas foram de alta qualidade, pontos fortes deste estudo. Por fim, à semelhança de todos os estudos com desenho retrospectivo, existem possíveis limitações, tais como vieses de confusão e informação. No entanto, não acreditamos que isso tenha afetado a validade de nossos achados. Os dados usados foram avaliados e documentados principalmente durante o período de hospitalização dos pacientes.
Outro ponto que deve ser enfatizado é que nossos resultados não puderam ser generalizados para a população ambulatorial. Os pacientes internados com PAC possuem características próprias, sendo a idade mais avançada uma das mais importantes. A média de idade dos pacientes em nosso estudo foi de 67 anos, e apenas 24 pacientes tinham menos de 40 anos. Dados da literatura sugerem que o desempenho do PSI é ruim em pacientes mais jovens,(25,26) e é possível que o mesmo ocorra com o ICC. Como no presente estudo houve apenas uma morte entre os pacientes mais jovens, não foi possível fazer esse tipo de avaliação.
Em suma, o presente estudo mostrou que o ICC, em comparação com o PSI e o CURB-65, é um preditor melhor de mortalidade hospitalar por qualquer causa em pacientes com PAC. O uso do ICC no PS pode contribuir para reduzir a mortalidade de pacientes com PAC.
CONTRIBUIÇÕES DOS AUTORES LFB: concepção e desenho do estudo, interpretação dos dados, redação e revisão do manuscrito e aprovação da versão final; LPD e SCF: concepção e desenho do estudo, redação e revisão do manuscrito e aprovação da versão final.
REFERÊNCIAS
- World Health Organization [homepage on the Internet]. Geneva: World Health Organization; [cited 2020 Sep 1]. Causes of death: Ten leading causes of death, 2012. Available from: http://apps.who.int/gho/data/view.wrapper.MGHEMORTCAUSE10?lang=en.
- Marrie TJ, Huang JQ. Epidemiology of community-acquired pneumonia in Edmonton, Alberta: an emergency department-based study. Can Respir J. 2005;12(3):139-142. https://doi.org/10.1155/2005/672501
- File TM Jr, Marrie TJ. Burden of community-acquired pneumonia in North American adults. Postgrad Med. 2010;122(2):130-141. https://doi.org/10.3810/pgm.2010.03.2130
- Myint PK, Sankaran P, Musonda P, Subramanian DN, Ruffell H, Smith AC, et al. Performance of CURB-65 and CURB-age in community-acquired pneumonia. Int J Clin Pract. 2009;63(9):1345-1350. https://doi.org/10.1111/j.1742-1241.2009.02147.x
- Neill AM, Martin IR, Weir R, Anderson R, Chereshsky A, Epton MJ, et al. Community acquired pneumonia: aetiology and usefulness of severity criteria on admission. Thorax. 1996;51(10):1010-1016. https://doi.org/10.1136/thx.51.10.1010
- Woodhead MA, Macfarlane JT, McCracken JS, Rose DH, Finch RG. Prospective study of the aetiology and outcome of pneumonia in the community. Lancet. 1987;1(8534):671-674. https://doi.org/10.1016/S0140-6736(87)90430-2
- Mandell LA, Wunderink RG, Anzueto A, Bartlett JG, Campbell GD, Dean NC, et al. Infectious Diseases Society of America/American Thoracic Society consensus guidelines on the management of community-acquired pneumonia in adults. Clin Infect Dis. 2007;44 Suppl 2(Suppl 2):S27-S72. https://doi.org/10.1086/511159
- British Thoracic Society Standards of Care Committee. BTS Guidelines for the Management of Community Acquired Pneumonia in Adults. Thorax. 2001;56 Suppl 4(Suppl 4):IV1-IV64. https://doi.org/10.1136/thx.56.suppl_4.iv1
- Corrêa Rde A, Lundgren FL, Pereira-Silva JL, Frare e Silva RL, Cardoso AP, Lemos AC, et al. Brazilian guidelines for community-acquired pneumonia in immunocompetent adults - 2009. J Bras Pneumol. 2009;35(6):574-601. https://doi.org/10.1590/S1806-37132009000600011
- Lim WS, van der Eerden MM, Laing R, Boersma WG, Karalus N, Town GI, et al. Defining community acquired pneumonia severity on presentation to hospital: an international derivation and validation study. Thorax. 2003;58(5):377-382. https://doi.org/10.1136/thorax.58.5.377
- Fine MJ, Auble TE, Yealy DM, Hanusa BH, Weissfeld LA, Singer DE, et al. A prediction rule to identify low-risk patients with community-acquired pneumonia. N Engl J Med. 1997;336(4):243-250. https://doi.org/10.1056/NEJM199701233360402
- Kwok CS, Loke YK, Woo K, Myint PK. Risk prediction models for mortality in community-acquired pneumonia: a systematic review. Biomed Res Int. 2013;2013:504136. https://doi.org/10.1155/2013/504136
- Lu KJ, Kearney LG, Ord M, Jones E, Burrell LM, Srivastava PM. Age adjusted Charlson Co-morbidity Index is an independent predictor of mortality over long-term follow-up in infective endocarditis. Int J Cardiol. 2013;168(6):5243-5248. https://doi.org/10.1016/j.ijcard.2013.08.023
- Martins M, Blais R. Evaluation of comorbidity indices for inpatient mortality prediction models. J Clin Epidemiol. 2006;59(7):665-669. https://doi.org/10.1016/j.jclinepi.2005.11.017
- Charlson ME, Pompei P, Ales KL, MacKenzie CR. A new method of classifying prognostic comorbidity in longitudinal studies: development and validation. J Chronic Dis. 1987;40(5):373-383. https://doi.org/10.1016/0021-9681(87)90171-8
- Sundararajan V, Henderson T, Perry C, Muggivan A, Quan H, Ghali WA. New ICD-10 version of the Charlson comorbidity index predicted in-hospital mortality. J Clin Epidemiol. 2004;57(12):1288-1294. https://doi.org/10.1016/j.jclinepi.2004.03.012
- Zavascki AP, Fuchs SC. The need for reappraisal of AIDS score weight of Charlson comorbidity index. J Clin Epidemiol. 2007;60(9):867-868. https://doi.org/10.1016/j.jclinepi.2006.11.004
- Bahlis LF, Diogo LP, Kuchenbecker RS, Fuchs SC. Clinical, epidemiological, and etiological profile of inpatients with community-acquired pneumonia in a public hospital in the interior of Brazil. J Bras Pneumol. 2018;44(4):261-266. https://doi.org/10.1590/s1806-37562017000000434
- Hanley JA, McNeil BJ. The meaning and use of the area under a receiver operating characteristic (ROC) curve. Radiology. 1982;143(1):29-36. https://doi.org/10.1148/radiology.143.1.7063747
- Wesemann T, Nüllmann H, Pflug MA, Heppner HJ, Pientka L, Thiem U. Pneumonia severity, comorbidity and 1-year mortality in predominantly older adults with community-acquired pneumonia: a cohort study. BMC Infect Dis. 2015;15:2. https://doi.org/10.1186/s12879-014-0730-x
- Salluh JI, Lisboa T, Bozza FA, Soares M, Póvoa P. Management of severe community-acquired pneumonia: a survey on the attitudes of 468 physicians in Iberia and South America. J Crit Care. 2014;29(5):743-747. https://doi.org/10.1016/j.jcrc.2014.05.019
- Rabello L, Conceição C, Ebecken K, Lisboa T, Bozza FA, Soares M, et al. Management of severe community-acquired pneumonia in Brazil: a secondary analysis of an international survey. Rev Bras Ter Intensiva. 2015;27(1):57-63. https://doi.org/10.5935/0103-507X.20150010
- Grendar J, Shaheen AA, Myers RP, Parker R, Vollmer CM Jr, Ball CG, et al. Predicting in-hospital mortality in patients undergoing complex gastrointestinal surgery: determining the optimal risk adjustment method. Arch Surg. 2012;147(2):126-135. https://doi.org/10.1001/archsurg.2011.296
- Budweiser S, Harlacher M, Pfeifer M, Jörres RA. Co-morbidities and hyperinflation are independent risk factors of all-cause mortality in very severe COPD. COPD. 2014;11(4):388-400. https://doi.org/10.3109/15412555.2013.836174
- Prina E, Ranzani OT, Torres A. Community-acquired pneumonia. Lancet. 2015;386(9998):1097-1108. https://doi.org/10.1016/S0140-6736(15)60733-4
- Marti C, Garin N, Grosgurin O, Poncet A, Combescure C, Carballo S, et al. Prediction of severe community-acquired pneumonia: a systematic review and meta-analysis. Crit Care. 2012;16(4):R141. https://doi.org/10.1186/cc11447